Multi-target model visual tracking method based on cost-sensitive three-way decision-making
A multi-objective model and cost-sensitive technology, applied in the field of surveillance video intelligent analysis, can solve problems such as lack of multi-features, multi-decision types, poor accuracy and robustness, etc., achieve accurate and robust tracking effects, and improve The effect of accuracy
- Summary
- Abstract
- Description
- Claims
- Application Information
AI Technical Summary
Problems solved by technology
Method used
Image
Examples
Embodiment
[0048] In order to make the object of the present invention, the technical solution and advantages more clearly understood, the following combined embodiments, as specific as Figure 1 Algorithm flowchart shown, the present invention is further elaborated in detail. It should be understood that the specific embodiments described herein are only used to explain the present invention, but do not limit the present invention.
[0049] Step 1: Establish multiple target models with different update strategies, which are described as follows: The target model should have the following characteristics: keep the characteristics of the tracked target unchanged, adapt to the appearance changes of the target in time, and be able to predict the future appearance changes of the target. Thus, the present invention first saves the target appearance of the initial frame, as a target feature that remains unchanged; secondly, the target appearance will be updated frame by frame, as a target feature ...
specific Embodiment approach
[0067] Figure 1 is an algorithm implementation flowchart of the present invention, the specific embodiments are as follows:
[0068] 1. Establish multiple target models with different update strategies and form a collection
[0069] 2. Use similarity learning model tracking to obtain multi-model candidate boxes ij for subsequent calculations of Articles 3 and 6;
[0070] 3. Calculate the number N of all candidate boxes that overlap in two pairs t ;
[0071] 4. Set a cost dictionary for each decision type, according to N t Calculate the cost of decision-making;
[0072] 5. Based on the cost-sensitive theory of three-branch decision-making, calculate the upper and lower boundaries of the three decision-making α and β according to the decision-making cost;
[0073] 6. According to the upper and lower boundary α and β, the candidate box obtained in step 2 is classified by using the convolutional neural network discriminator;
[0074] 7. The candidate box with the highest confidence ...
PUM
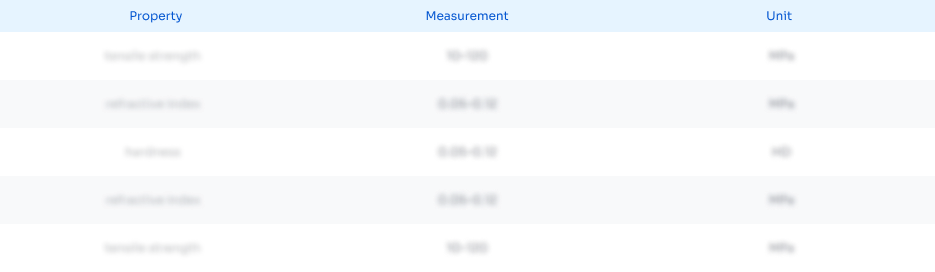
Abstract
Description
Claims
Application Information
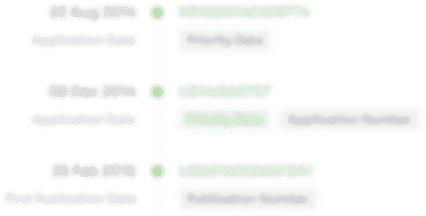
- R&D
- Intellectual Property
- Life Sciences
- Materials
- Tech Scout
- Unparalleled Data Quality
- Higher Quality Content
- 60% Fewer Hallucinations
Browse by: Latest US Patents, China's latest patents, Technical Efficacy Thesaurus, Application Domain, Technology Topic, Popular Technical Reports.
© 2025 PatSnap. All rights reserved.Legal|Privacy policy|Modern Slavery Act Transparency Statement|Sitemap|About US| Contact US: help@patsnap.com