Single-person attitude estimation method based on multistage prediction feature enhanced convolutional neural network
A convolutional neural network and feature enhancement technology, applied in the field of computer vision, can solve the problems of sharp increase in the number of model parameters, unfavorable learning opportunities for skeleton point learning, and indistinguishable degree of difficulty of human pose skeleton points, so as to overcome the problems of human pose skeleton. The point feature representation is inaccurate, the accuracy and speed are improved, and the skeleton point feature is fine.
- Summary
- Abstract
- Description
- Claims
- Application Information
AI Technical Summary
Problems solved by technology
Method used
Image
Examples
Embodiment Construction
[0028] The present invention will be further described in detail below in conjunction with the accompanying drawings and specific implementation examples.
[0029] refer to figure 1 , the present invention comprises the following steps:
[0030] (1) Obtain training set and test set:
[0031] Randomly select M image samples with real labels from the single-person pose estimation data set to form a training set, and select N image samples with real labels to form a test set, in which the number of categories of human skeleton points contained in each label is is P, the number of human skeleton points in each category is 1, M=2000, N=10000, P=14;
[0032] (2) Classify the skeleton points of the human body:
[0033] (2a) The test set is used as the input of the multi-stage feature fusion single-person pose estimation model. In this embodiment, the Hourglass model with high accuracy at this stage is used to predict the position of each type of human skeleton point for each image...
PUM
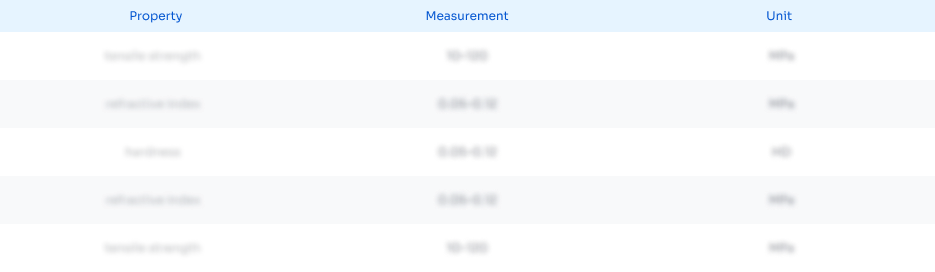
Abstract
Description
Claims
Application Information
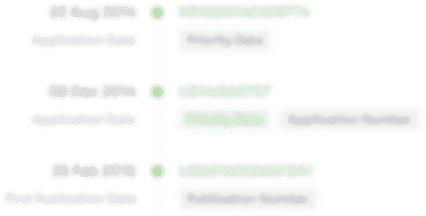
- R&D Engineer
- R&D Manager
- IP Professional
- Industry Leading Data Capabilities
- Powerful AI technology
- Patent DNA Extraction
Browse by: Latest US Patents, China's latest patents, Technical Efficacy Thesaurus, Application Domain, Technology Topic, Popular Technical Reports.
© 2024 PatSnap. All rights reserved.Legal|Privacy policy|Modern Slavery Act Transparency Statement|Sitemap|About US| Contact US: help@patsnap.com