Multi-label Image Classification Method Based on Manifold Learning and Gradient Boosting Model
A technology of manifold learning and classification method, applied in the field of two-stage partial multi-label learning, can solve problems such as limited prediction performance, and achieve the effect of improving prediction performance, excellent performance, and realizing disambiguation
- Summary
- Abstract
- Description
- Claims
- Application Information
AI Technical Summary
Problems solved by technology
Method used
Image
Examples
Embodiment Construction
[0064] The technical solution of the present invention will be further described in conjunction with specific implementation and examples.
[0065] Such as figure 1 , specific embodiments of the present invention and its implementation process are as follows:
[0066] In the first stage, label disambiguation is performed first, including step 1 and step 2:
[0067] step 1:
[0068] First, from the pre-specified training data set Build a weighted graph in Among them, V represents the collection of picture feature vectors, V={x i |1≤i≤n},x i Represents the feature vector of the i-th picture, i represents the ordinal number of the picture, and n represents the training data set The total number of pictures in; E represents the set of connections between every two pictures, E={(x i ,x j )|i≠j,x j ∈kNN(x i )}, kNN(x i ) represents the feature vector x to the i-th picture i The set of feature vectors of the first k pictures with the closest Euclidean distance, (x i ...
PUM
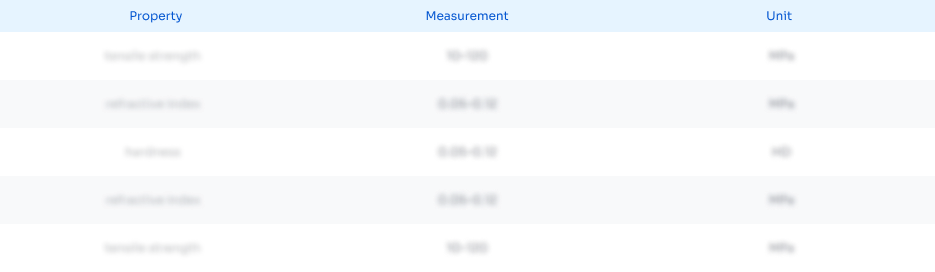
Abstract
Description
Claims
Application Information
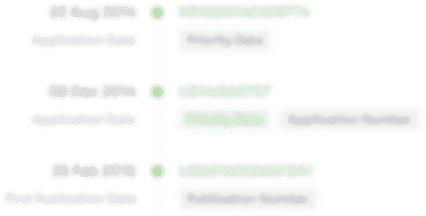
- R&D Engineer
- R&D Manager
- IP Professional
- Industry Leading Data Capabilities
- Powerful AI technology
- Patent DNA Extraction
Browse by: Latest US Patents, China's latest patents, Technical Efficacy Thesaurus, Application Domain, Technology Topic, Popular Technical Reports.
© 2024 PatSnap. All rights reserved.Legal|Privacy policy|Modern Slavery Act Transparency Statement|Sitemap|About US| Contact US: help@patsnap.com