Unsupervised learning of semantic audio representations
A technology of anchor audio and audio clips, applied in the field of unsupervised learning of semantic audio representation, which can solve the problems of expensive manual tag generation and lack of tags with sound content
- Summary
- Abstract
- Description
- Claims
- Application Information
AI Technical Summary
Problems solved by technology
Method used
Image
Examples
Embodiment Construction
[0018] Examples of methods and systems are described herein. It should be understood that the words "exemplary," "example," and "illustrative" are used herein to mean "serving as an example, instance, or illustration." Any embodiment or feature described herein as "exemplary," "example" or "illustrative" is not necessarily to be construed as preferred or advantageous over other embodiments or features. Furthermore, the exemplary embodiments described herein are not meant to be limiting. It should be readily appreciated that certain aspects of the disclosed systems and methods can be arranged and combined in many different configurations.
[0019] I. overview
[0020] Audio recordings can include various non-speech sounds. These non-speech sounds may include noises related to the operation of machinery, weather, human or animal motion, sirens or other warning sounds, barking or other sounds produced by animals, or other sounds. Such sounds may provide an indication of whe...
PUM
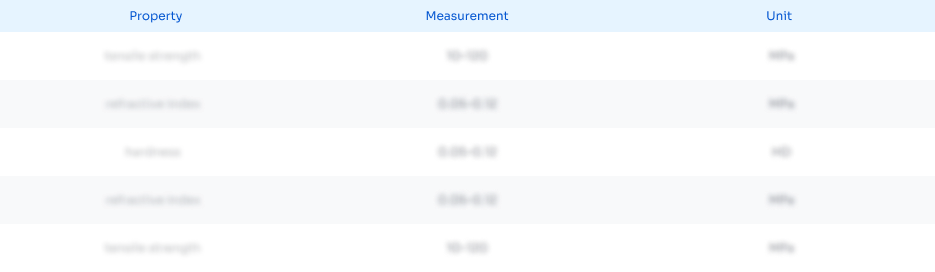
Abstract
Description
Claims
Application Information
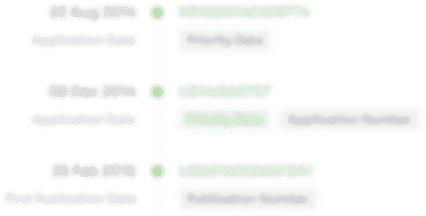
- R&D Engineer
- R&D Manager
- IP Professional
- Industry Leading Data Capabilities
- Powerful AI technology
- Patent DNA Extraction
Browse by: Latest US Patents, China's latest patents, Technical Efficacy Thesaurus, Application Domain, Technology Topic, Popular Technical Reports.
© 2024 PatSnap. All rights reserved.Legal|Privacy policy|Modern Slavery Act Transparency Statement|Sitemap|About US| Contact US: help@patsnap.com