Behavior recognition method based on graph convolution and capsule neural network
A convolutional neural network and neural network technology, applied in neural learning methods, biological neural network models, character and pattern recognition, etc., can solve problems such as inability to draw inferences from one example, and the relative relationship between component orientation and space is not important
- Summary
- Abstract
- Description
- Claims
- Application Information
AI Technical Summary
Problems solved by technology
Method used
Image
Examples
Embodiment Construction
[0111] In order to make the object, technical solution and advantages of the present invention clearer, the present invention will be further described in detail below in conjunction with the accompanying drawings and embodiments. It should be understood that the specific embodiments described here are only used to explain the present invention, not to limit the present invention. In addition, the technical features involved in the various embodiments of the present invention described below can be combined with each other as long as they do not constitute a conflict with each other.
[0112] The present invention introduces the NTU RGB+D data set as a multi-frame human continuous action image;
[0113] Combine below Figure 1 to Figure 3 The specific embodiment of the present invention is introduced as a behavior recognition method based on graph convolution and capsule neural network, which specifically includes the following steps:
[0114] Step 1: Collect multiple frames...
PUM
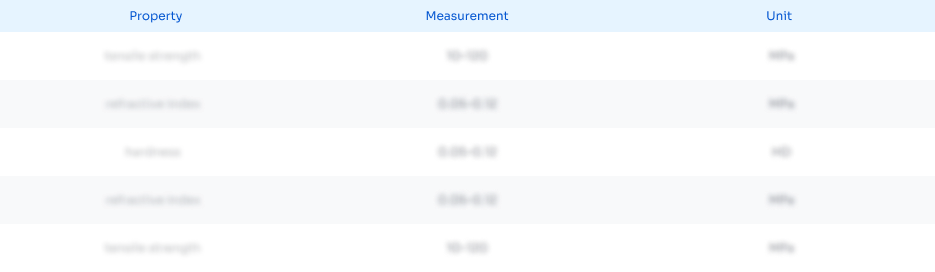
Abstract
Description
Claims
Application Information
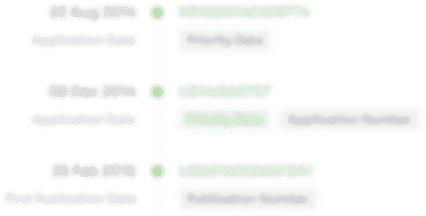
- R&D Engineer
- R&D Manager
- IP Professional
- Industry Leading Data Capabilities
- Powerful AI technology
- Patent DNA Extraction
Browse by: Latest US Patents, China's latest patents, Technical Efficacy Thesaurus, Application Domain, Technology Topic.
© 2024 PatSnap. All rights reserved.Legal|Privacy policy|Modern Slavery Act Transparency Statement|Sitemap