A Generic Perturbation Generation Method Based on Generative Adversarial Networks
A network, adversarial sample technology, applied in the field of deep learning, can solve the problem of limited rights of attackers
- Summary
- Abstract
- Description
- Claims
- Application Information
AI Technical Summary
Problems solved by technology
Method used
Image
Examples
Embodiment Construction
[0017] In order to facilitate those skilled in the art to understand and implement the present invention, the present invention will be described in further detail below in conjunction with the accompanying drawings and embodiments. It should be understood that the implementation examples described here are only used to illustrate and explain the present invention, not for limit the invention.
[0018] The general disturbance generation method under the black-box scenario based on Generative Adversarial Net (GAN) provided by the present invention includes the general disturbance generation network to realize the function mapping from random noise pictures to general disturbances, and the adversarial sample discrimination network to predict that the network input is The probability and objective function of real samples are used to train the generation network and discriminant network to improve the success rate of adversarial sample attacks; the general perturbation generation ...
PUM
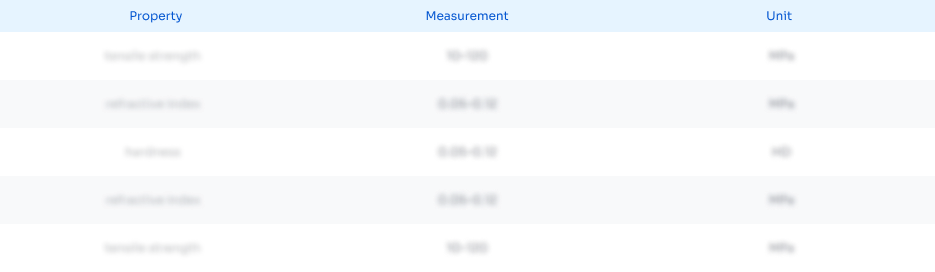
Abstract
Description
Claims
Application Information
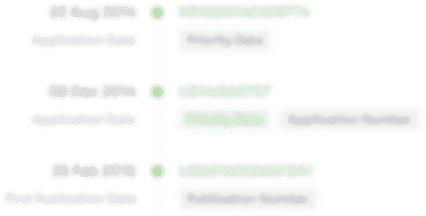
- R&D Engineer
- R&D Manager
- IP Professional
- Industry Leading Data Capabilities
- Powerful AI technology
- Patent DNA Extraction
Browse by: Latest US Patents, China's latest patents, Technical Efficacy Thesaurus, Application Domain, Technology Topic, Popular Technical Reports.
© 2024 PatSnap. All rights reserved.Legal|Privacy policy|Modern Slavery Act Transparency Statement|Sitemap|About US| Contact US: help@patsnap.com