Anti-attack method based on training set data
A training set and data technology, applied in the fields of instruments, character and pattern recognition, computer components, etc., can solve the problem of unconvincing linear assumptions, and achieve the effect of small disturbance
- Summary
- Abstract
- Description
- Claims
- Application Information
AI Technical Summary
Problems solved by technology
Method used
Image
Examples
Embodiment Construction
[0039] The present invention will be further described below in conjunction with the accompanying drawings and embodiments, but not as a basis for limiting the present invention.
[0040] Such as figure 1 As shown, it is an overall flowchart of an adversarial attack method based on training set data in the present invention, and YOLO-v3 is selected as the target model, which specifically includes the following steps:
[0041] Step 1, use the image collection Img composed of the VOC large-scale image classification dataset to train the detection model:
[0042]
[0043] where x i represents an image, N d Indicates the total number of images in the image collection Img;
[0044] Build a collection IMG of image sets (consisting of Img), where each image x k Corresponding detection frame T k :
[0045] T k ={(q 11 ,p 11 ,q 21 ,p 21 , l 1 )...(q 1i ,p 1i ,q 2i ,p 2i , l i )}, i=1,2,...,R n
[0046] Among them, (q 11 ,p 11 ,q 21 ,p 21 ) represents the coord...
PUM
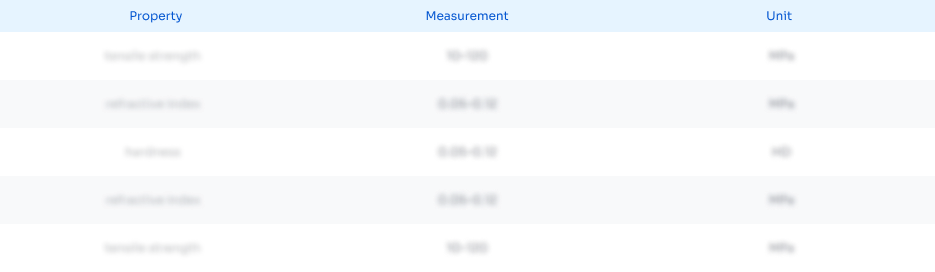
Abstract
Description
Claims
Application Information
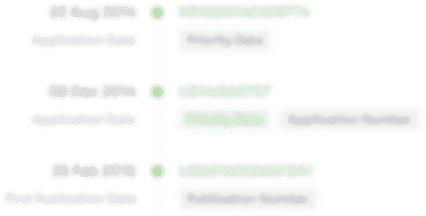
- R&D
- Intellectual Property
- Life Sciences
- Materials
- Tech Scout
- Unparalleled Data Quality
- Higher Quality Content
- 60% Fewer Hallucinations
Browse by: Latest US Patents, China's latest patents, Technical Efficacy Thesaurus, Application Domain, Technology Topic, Popular Technical Reports.
© 2025 PatSnap. All rights reserved.Legal|Privacy policy|Modern Slavery Act Transparency Statement|Sitemap|About US| Contact US: help@patsnap.com