Small sample learning method for external memory and meta-learning based on attention guidance
A learning method and attention technology, applied in the field of small sample learning of external memory and meta-learning, can solve the problem of insufficient number of samples
- Summary
- Abstract
- Description
- Claims
- Application Information
AI Technical Summary
Problems solved by technology
Method used
Image
Examples
Embodiment
[0097] A few-shot learning method based on attention-guided external memory and meta-learning, including the following steps:
[0098] Input: optimized network parameters Θ={θ 1 , θ 2 , θ 3 , φ}, the supporting data set S={x under the new task j ,y j} and queryset
[0099] Output: the prediction results of the query set
[0100] S1. For the supporting data set and query set data, calculate their characteristics and key index values as follows:
[0101]
[0102] S2. For category c in the classifier, perform the following calculations:
[0103]
[0104]
[0105]
[0106] S3. Construct the overall classifier as follows:
[0107] W=[w 1 ,...,w c ,...,w C ]
[0108] b=[b 1 ,...,b c ,...,b C ];
[0109] S4. Calculate attention weight ω q,i and the reference value v obtained from the memory mechanism according to the attention q :
[0110]
[0111]
[0112] S5. For the query set, calculate its characteristic expression as follows:
[0113] ...
PUM
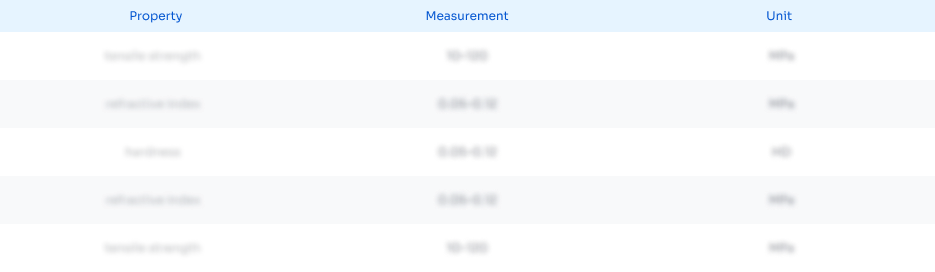
Abstract
Description
Claims
Application Information
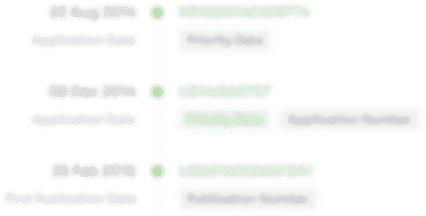
- Generate Ideas
- Intellectual Property
- Life Sciences
- Materials
- Tech Scout
- Unparalleled Data Quality
- Higher Quality Content
- 60% Fewer Hallucinations
Browse by: Latest US Patents, China's latest patents, Technical Efficacy Thesaurus, Application Domain, Technology Topic, Popular Technical Reports.
© 2025 PatSnap. All rights reserved.Legal|Privacy policy|Modern Slavery Act Transparency Statement|Sitemap|About US| Contact US: help@patsnap.com