A Knowledge Graph Representation Learning Method Fused with Entity Description and Type
A knowledge graph and learning method technology, applied in character and pattern recognition, instruments, semantic analysis, etc., can solve the problems of low degree of type information fusion and single fusion method, so as to reduce the degree of ambiguity, improve semantic information, and improve accuracy Effect
- Summary
- Abstract
- Description
- Claims
- Application Information
AI Technical Summary
Problems solved by technology
Method used
Image
Examples
Embodiment Construction
[0063] example, as figure 1 It is the overall flow chart of a knowledge graph that integrates entity descriptions and types of the present invention to represent the learning method; it specifically includes:
[0064] Step S1: utilize the translation model to obtain the embedding of triplet entities, and treat the relationship in the triplet as a translation operation between the head entity and the tail entity, and obtain the numerical vector representation of each triplet entity and relationship;
[0065] 1) TransE model gets triple embedding
[0066] The flow chart of TransE method to obtain entity embedding is as follows figure 2 shown.
[0067] First, the vector representations of the triplet head entity, relation, and tail entity are randomly generated, denoted as h, r, and t, respectively.
[0068] Secondly, according to the idea that the relationship is the translation operation between the head entity and the tail entity, the negative sample data is randomly gener...
PUM
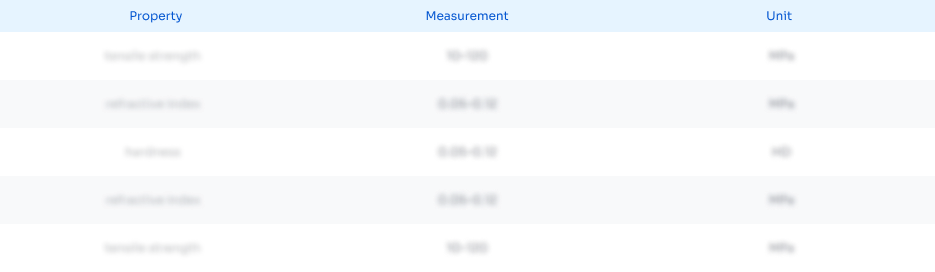
Abstract
Description
Claims
Application Information
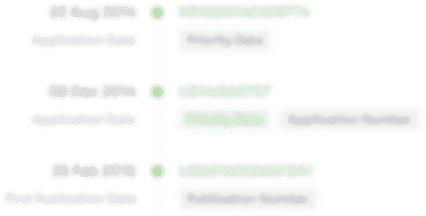
- R&D Engineer
- R&D Manager
- IP Professional
- Industry Leading Data Capabilities
- Powerful AI technology
- Patent DNA Extraction
Browse by: Latest US Patents, China's latest patents, Technical Efficacy Thesaurus, Application Domain, Technology Topic, Popular Technical Reports.
© 2024 PatSnap. All rights reserved.Legal|Privacy policy|Modern Slavery Act Transparency Statement|Sitemap|About US| Contact US: help@patsnap.com