Video behavior recognition method based on spatial-temporal feature fusion deep learning network
A technology of deep learning network and spatio-temporal features, which is applied in the field of video behavior recognition based on spatio-temporal feature fusion deep learning network, can solve problems such as confusion, insufficiency, complex network structure training volume, etc., and achieve the goal of improving influence and recognition accuracy Effect
- Summary
- Abstract
- Description
- Claims
- Application Information
AI Technical Summary
Problems solved by technology
Method used
Image
Examples
Embodiment Construction
[0063] In order to make the object, technical solution and advantages of the present invention clearer, the present invention will be further described in detail below in conjunction with the accompanying drawings and embodiments. It should be understood that the specific embodiments described here are only used to explain the present invention, not to limit the present invention.
[0064] A kind of video behavior recognition method based on spatio-temporal feature fusion deep learning network, its characteristic comprises the following steps:
[0065] A kind of video behavior recognition method based on spatio-temporal feature fusion deep learning network, its characteristic comprises the following steps:
[0066] (1) Expand the data set through three methods: horizontal mirror inversion, small-angle rotation, and cropping. The small-angle rotation is clockwise rotation of 30°, 15°, -15° and -30° respectively, and two independent networks are used The time and space informat...
PUM
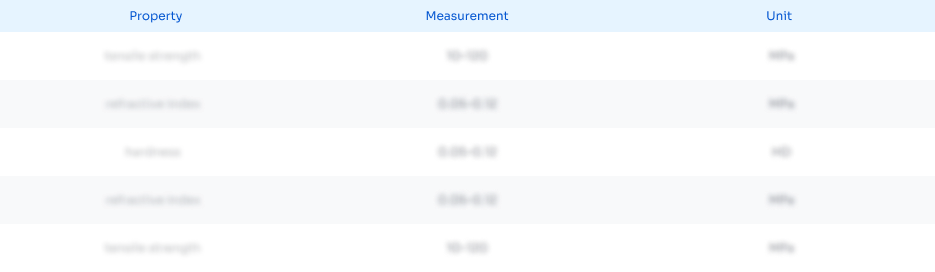
Abstract
Description
Claims
Application Information
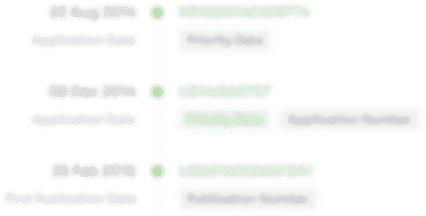
- R&D Engineer
- R&D Manager
- IP Professional
- Industry Leading Data Capabilities
- Powerful AI technology
- Patent DNA Extraction
Browse by: Latest US Patents, China's latest patents, Technical Efficacy Thesaurus, Application Domain, Technology Topic, Popular Technical Reports.
© 2024 PatSnap. All rights reserved.Legal|Privacy policy|Modern Slavery Act Transparency Statement|Sitemap|About US| Contact US: help@patsnap.com