Distributed multi-task learning privacy protection method and system against inference attack
A multi-task learning and privacy protection technology, applied in the field of distributed multi-task learning privacy protection, to overcome the high computational cost and ensure data privacy.
- Summary
- Abstract
- Description
- Claims
- Application Information
AI Technical Summary
Problems solved by technology
Method used
Image
Examples
Embodiment Construction
[0093] The present invention will be further described in detail below in conjunction with the accompanying drawings and embodiments.
[0094]The invention designs a distributed multi-task learning privacy protection system against reasoning attacks, which is composed of a key generation center, a central server, task nodes and model users. The key generation center is mainly responsible for key generation, and distributes keys for the central server and each task node. In this system, the key generation center is the only trusted entity; the central server is mainly responsible for managing the parameter sharing part uploaded by each task node The product of the matrix represented by the training sample, and provide a certain amount of computing power to be responsible for updating the product aggregation data of each task node. In this system, the central server is a semi-trusted entity, which can correctly manage and calculate the data for model training, but It will also i...
PUM
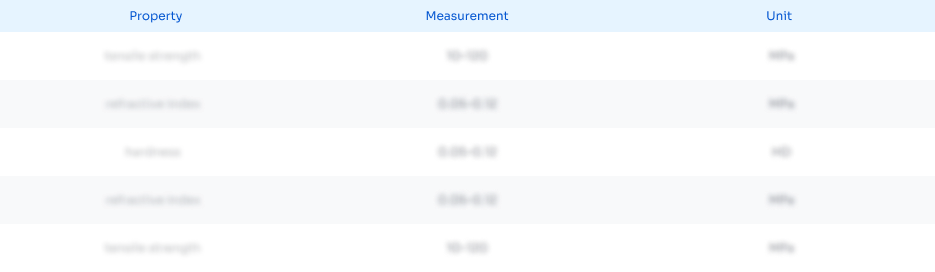
Abstract
Description
Claims
Application Information
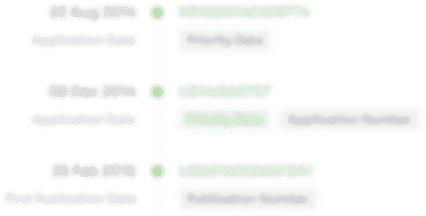
- R&D Engineer
- R&D Manager
- IP Professional
- Industry Leading Data Capabilities
- Powerful AI technology
- Patent DNA Extraction
Browse by: Latest US Patents, China's latest patents, Technical Efficacy Thesaurus, Application Domain, Technology Topic, Popular Technical Reports.
© 2024 PatSnap. All rights reserved.Legal|Privacy policy|Modern Slavery Act Transparency Statement|Sitemap|About US| Contact US: help@patsnap.com