Prediction method of urban congestion propagation mode based on cyclic autoregression model
A technology of autoregressive model and propagation mode, applied in the field of intelligent transportation engineering, can solve the problems of lack of dynamic expression ability of congestion propagation, inability to express temporal and spatial evolution ability of congestion propagation, and only consideration
- Summary
- Abstract
- Description
- Claims
- Application Information
AI Technical Summary
Problems solved by technology
Method used
Image
Examples
Embodiment Construction
[0063] The present invention will be further described below in conjunction with specific examples, but the present invention is not limited to these specific implementations. Those skilled in the art will realize that the present invention covers all alternatives, modifications and equivalents as may be included within the scope of the claims.
[0064] This embodiment provides a method for predicting urban congestion propagation patterns based on cyclic autoregressive models, see figure 1 , the specific steps are as follows:
[0065] S1. Using the topological connection relationship of the urban road network, generate a road section connection relationship graph N;
[0066] S2. Select historical data for a long period of time, and construct a time-series knowledge graph G of congestion propagation based on the link connection graph N, where the long period of time is at least 1 month;
[0067] S3. Train the cyclic autoregressive model, learn the congestion propagation mode ...
PUM
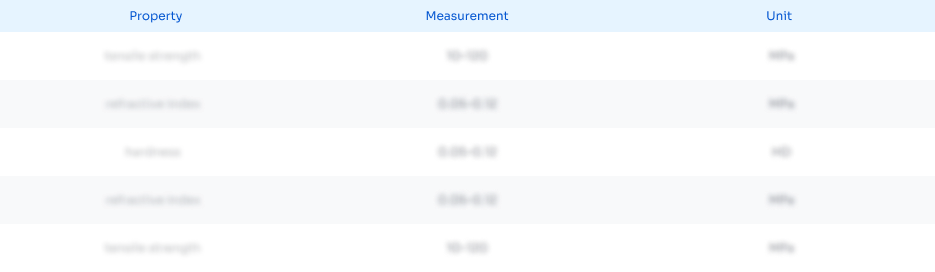
Abstract
Description
Claims
Application Information
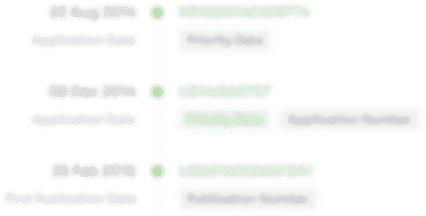
- R&D
- Intellectual Property
- Life Sciences
- Materials
- Tech Scout
- Unparalleled Data Quality
- Higher Quality Content
- 60% Fewer Hallucinations
Browse by: Latest US Patents, China's latest patents, Technical Efficacy Thesaurus, Application Domain, Technology Topic, Popular Technical Reports.
© 2025 PatSnap. All rights reserved.Legal|Privacy policy|Modern Slavery Act Transparency Statement|Sitemap|About US| Contact US: help@patsnap.com