Adaptive target detection method based on lightweight residual learning and deconvolution cascading
A target detection and lightweight technology, applied in character and pattern recognition, instruments, biological neural network models, etc., can solve problems such as large amount of calculation, poor algorithm robustness, and low target detection accuracy, and achieve fast and accurate identification and positioning , high precision and robustness, to meet the effect of real-time detection
- Summary
- Abstract
- Description
- Claims
- Application Information
AI Technical Summary
Problems solved by technology
Method used
Image
Examples
Embodiment Construction
[0020] Embodiments of the present invention are described in detail below, examples of which are shown in the drawings, wherein the same or similar reference numerals designate the same or similar elements or elements having the same or similar functions throughout. The embodiments described below by referring to the figures are exemplary only for explaining the present invention and should not be construed as limiting the present invention.
[0021] Such as figure 1 As shown, according to the present invention, an adaptive target detection method based on lightweight residual learning and deconvolution cascading includes the following steps:
[0022] S1: collect data through image acquisition equipment, and obtain image training data sets and test data sets;
[0023] S1.1: Expand the dataset by preprocessing the samples in the dataset by cropping, flipping, rotating, and scaling;
[0024] S1.2: Extract the positive and negative samples in each image, mark the positive sampl...
PUM
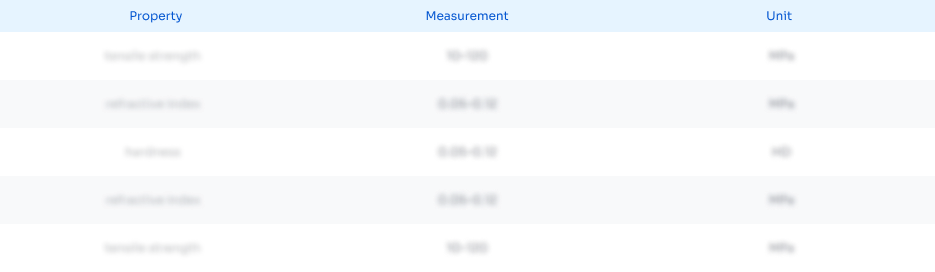
Abstract
Description
Claims
Application Information
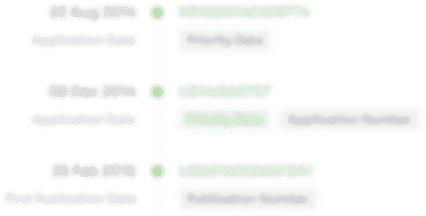
- R&D Engineer
- R&D Manager
- IP Professional
- Industry Leading Data Capabilities
- Powerful AI technology
- Patent DNA Extraction
Browse by: Latest US Patents, China's latest patents, Technical Efficacy Thesaurus, Application Domain, Technology Topic, Popular Technical Reports.
© 2024 PatSnap. All rights reserved.Legal|Privacy policy|Modern Slavery Act Transparency Statement|Sitemap|About US| Contact US: help@patsnap.com