A semi-supervised image classification method based on random area interpolation
A classification method, a semi-supervised technology, applied in neural learning methods, computer components, instruments, etc., to achieve good classification effect, easy classification, and good generalization performance
- Summary
- Abstract
- Description
- Claims
- Application Information
AI Technical Summary
Problems solved by technology
Method used
Image
Examples
Embodiment Construction
[0043] like figure 1 The semi -supervised image classification method based on the random area interpolation provided by this embodiment, including the following steps:
[0044] S1. Divide the training data and divide the real label image collection No real tag image collection Test data set details as follows:
[0045] First, the image is flipped horizontally at a probability of 0.5, and then fills the width and height of the image. Then the image is randomly cut into a pixel of 32X32, then the image pixels are reduced, and finally the albinization process is processed; as needed as needed;Classify image data, first divide image data into training data and test data sets Two categories; then divide the training data into two categories: real label image collection Collection with no real tag image The ratio is 1:50, that is, the training data is equal to A real label image is recorded as Right now A real label image is recorded as Right now
[0046] S2, from no real la...
PUM
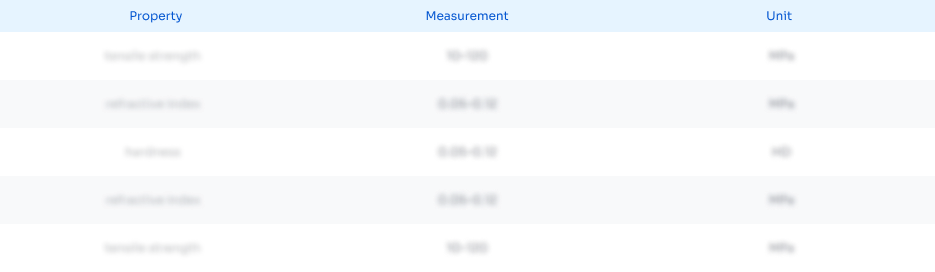
Abstract
Description
Claims
Application Information
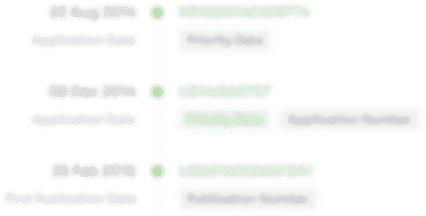
- R&D Engineer
- R&D Manager
- IP Professional
- Industry Leading Data Capabilities
- Powerful AI technology
- Patent DNA Extraction
Browse by: Latest US Patents, China's latest patents, Technical Efficacy Thesaurus, Application Domain, Technology Topic, Popular Technical Reports.
© 2024 PatSnap. All rights reserved.Legal|Privacy policy|Modern Slavery Act Transparency Statement|Sitemap|About US| Contact US: help@patsnap.com