Deep learning method for predicting binding site on antibody through sequence
A binding site and deep learning technology, applied in the field of antibody binding site prediction, can solve the problem of not considering interaction information, and achieve the effect of increasing interpretability and accurate prediction
- Summary
- Abstract
- Description
- Claims
- Application Information
AI Technical Summary
Problems solved by technology
Method used
Image
Examples
Embodiment 1
[0056] Such as figure 2 As shown, a deep learning method for predicting binding sites on antibodies through sequences, the method includes the following steps:
[0057] Obtain several hypervariable regions on the antibody, concatenate several hypervariable regions into a hypervariable region sequence, and add an unknown type of amino acid between different hypervariable region sequences as a distinguishing mark;
[0058] The features of each amino acid in the hypervariable region sequence include word embedding features and extra features; combining the word embedding features and extra features to obtain the final feature matrix, and inputting the feature matrix into the neural network model;
[0059] The neural network model uses a bidirectional long-short-term memory network and a transformer encoder to learn sequence information of hypervariable regions and interaction information between different hypervariable regions to predict antibody binding sites.
[0060] In a sp...
PUM
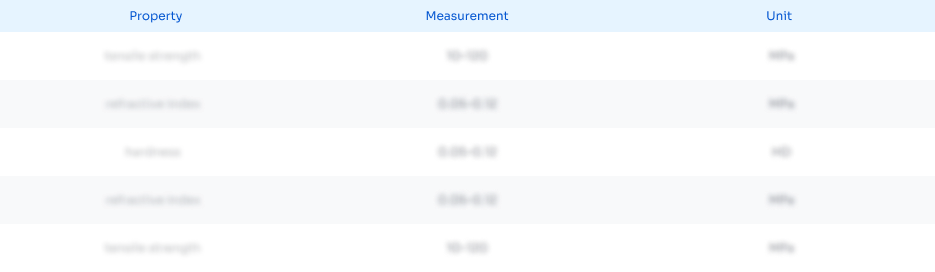
Abstract
Description
Claims
Application Information
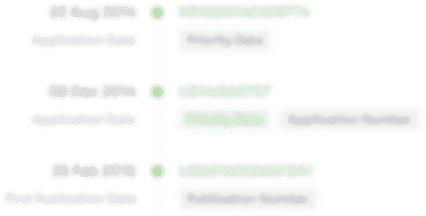
- R&D Engineer
- R&D Manager
- IP Professional
- Industry Leading Data Capabilities
- Powerful AI technology
- Patent DNA Extraction
Browse by: Latest US Patents, China's latest patents, Technical Efficacy Thesaurus, Application Domain, Technology Topic.
© 2024 PatSnap. All rights reserved.Legal|Privacy policy|Modern Slavery Act Transparency Statement|Sitemap