Multivariate time series multilayer space-time dependence modeling method based on deep learning
A multivariate time series and deep learning technology, applied in the field of deep learning, can solve problems such as the influence of different layers of features, achieve advanced performance, enhance interpretability and robustness, and improve accuracy
- Summary
- Abstract
- Description
- Claims
- Application Information
AI Technical Summary
Problems solved by technology
Method used
Image
Examples
Embodiment Construction
[0036] Following result accompanying drawing and specific embodiment are described in further detail to the present invention:
[0037] The purpose of the present invention is to solve the problem that the influence of different layer features on the prediction results is ignored in the process of current deep learning to capture spatiotemporal features of multivariate time series data to complete the prediction task.
[0038] Such as figure 1 , 2 , 3, 4, and 5, what the present invention provides is a multivariate time series multi-layer space-time dependent modeling method based on deep learning, comprising the following steps:
[0039] Step 1: Raw data is preprocessed, including the following steps:
[0040] (1) Perform z-score standardization on multivariate time series data;
[0041] (2) Introduce a sliding window to scroll multivariate time series data. The value in the sliding window is used as an attribute, and the value at the next moment is used as a label. The step...
PUM
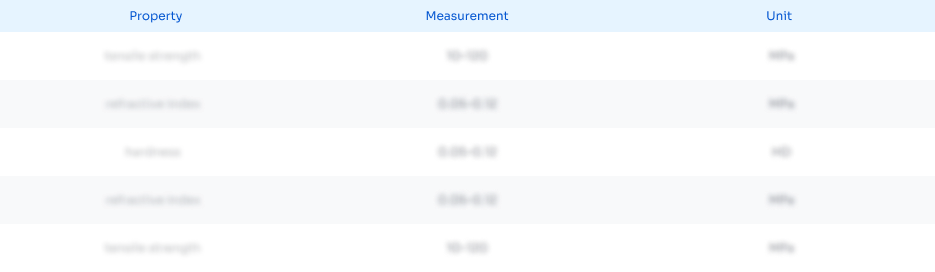
Abstract
Description
Claims
Application Information
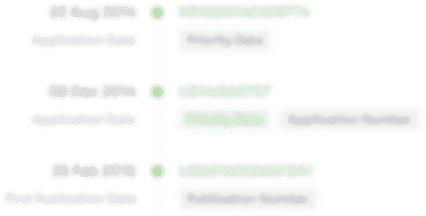
- R&D Engineer
- R&D Manager
- IP Professional
- Industry Leading Data Capabilities
- Powerful AI technology
- Patent DNA Extraction
Browse by: Latest US Patents, China's latest patents, Technical Efficacy Thesaurus, Application Domain, Technology Topic.
© 2024 PatSnap. All rights reserved.Legal|Privacy policy|Modern Slavery Act Transparency Statement|Sitemap