A Self-Supervised Monocular Depth Estimation Method Based on Deep Learning
A technology of depth estimation and deep learning, which is applied in the field of depth estimation and computer vision, can solve the problems of inaccurate parallax, insufficient exploration of geometric correlation, etc., and achieve the effect of improving accuracy
- Summary
- Abstract
- Description
- Claims
- Application Information
AI Technical Summary
Problems solved by technology
Method used
Image
Examples
Embodiment Construction
[0032] In order to make the objectives, technical solutions and advantages of the present invention clearer, the embodiments of the present invention are further described in detail below.
[0033] The embodiment of the present invention provides a self-supervised monocular depth estimation method based on deep learning, see figure 1 , the method includes the following steps:
[0034] 1. Building a monocular depth estimation network
[0035] On the original right view I r , using a monocular depth estimation network from the right view I r Learning right-to-left disparity maps in D l . The monocular depth estimation network adopts an encoder-decoder network structure with skip connections. The encoder network uses ResNet50 to extract features from the right view, and the decoder network consists of continuous deconvolution and skip connections to gradually The resolution of the feature map is restored to that of the input image. Disparity map D obtained from monocular de...
PUM
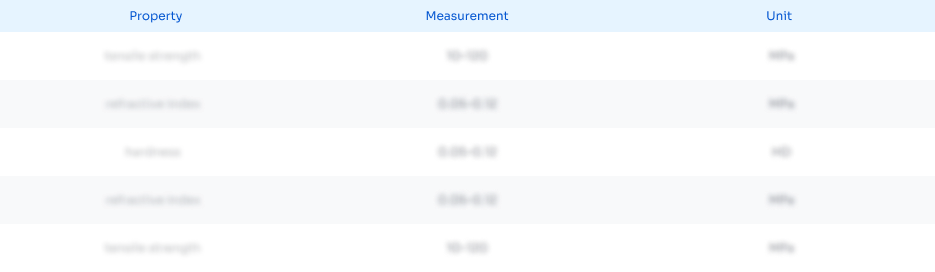
Abstract
Description
Claims
Application Information
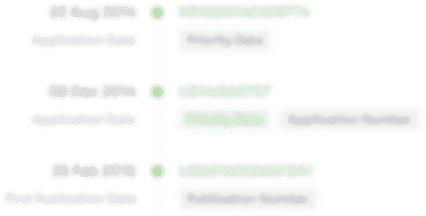
- R&D Engineer
- R&D Manager
- IP Professional
- Industry Leading Data Capabilities
- Powerful AI technology
- Patent DNA Extraction
Browse by: Latest US Patents, China's latest patents, Technical Efficacy Thesaurus, Application Domain, Technology Topic, Popular Technical Reports.
© 2024 PatSnap. All rights reserved.Legal|Privacy policy|Modern Slavery Act Transparency Statement|Sitemap|About US| Contact US: help@patsnap.com