Light field image depth estimation method based on deep convolutional neural network
A neural network and deep convolution technology, applied in the field of computer vision and artificial intelligence, can solve the problems of insufficient utilization of EPI texture structure, limited feature extraction ability, insufficient occlusion and noise processing ability, etc.
- Summary
- Abstract
- Description
- Claims
- Application Information
AI Technical Summary
Problems solved by technology
Method used
Image
Examples
Embodiment Construction
[0039] The present invention will be further described in detail below in conjunction with the accompanying drawings.
[0040] like figure 1 As shown, a method for estimating the depth of a light field image based on a deep convolutional neural network disclosed in the present invention includes the following steps:
[0041] Step 1: Extract the central subaperture image from the 4D light field data where (i C ,j C ) represents the viewing angle coordinates of the central sub-aperture image.
[0042] The 4D light field data is the decoded representation of the light field image collected by the light field camera, which is recorded as L:(i,j,k,l)→L(i,j,k,l), where (i,j) Represents the pixel index coordinates of the microlens image, (k,l) represents the index coordinates of the microlens center, L(i,j,k,l) represents the pixel through the microlens center (k,l) and the microlens image pixel (i, j) the radiation intensity of the light rays; the method of extracting the ce...
PUM
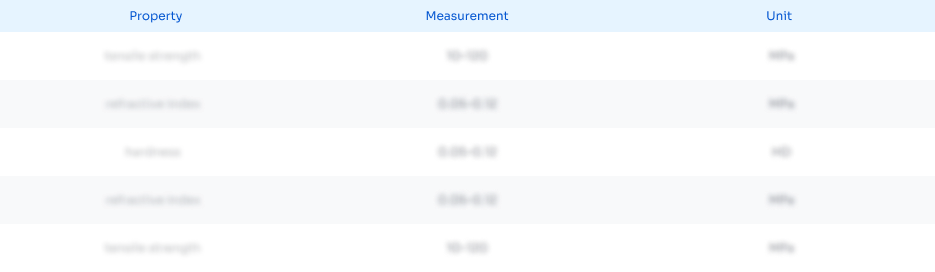
Abstract
Description
Claims
Application Information
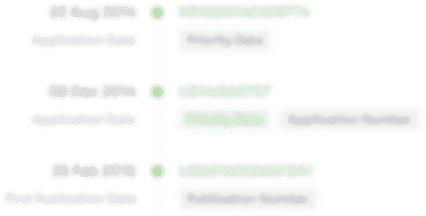
- R&D Engineer
- R&D Manager
- IP Professional
- Industry Leading Data Capabilities
- Powerful AI technology
- Patent DNA Extraction
Browse by: Latest US Patents, China's latest patents, Technical Efficacy Thesaurus, Application Domain, Technology Topic, Popular Technical Reports.
© 2024 PatSnap. All rights reserved.Legal|Privacy policy|Modern Slavery Act Transparency Statement|Sitemap|About US| Contact US: help@patsnap.com