A Multi-Core Processor-Single Graphics Processor Deep Reinforcement Learning Acceleration Method
A graphics processor and multi-core processor technology, applied in the computer field, can solve different and unrelated problems
- Summary
- Abstract
- Description
- Claims
- Application Information
AI Technical Summary
Problems solved by technology
Method used
Image
Examples
Embodiment Construction
[0023] In conjunction with the accompanying drawings, the technical solutions in the examples of the present invention are clearly and completely described below:
[0024] figure 1 Shown is the implementation process of the deep reinforcement learning acceleration method based on the multi-core CPU-GPU platform of the present invention, including the following steps:
[0025] 1. Allocate memory space for the CPU and GPU, among which three memory spaces are set on the CPU, one stores the experience information pool for network training; the other two memory spaces store the action network parameter θ and the evaluation network parameter ω respectively. Two memory spaces are allocated on the GPU to store local action network parameters θ - and locally evaluate the network parameter ω - . Except that the CPU and GPU can control the internal memory separately, the memory of the CPU and GPU can communicate through the PCIE bus, including two operations of reading and writing. U...
PUM
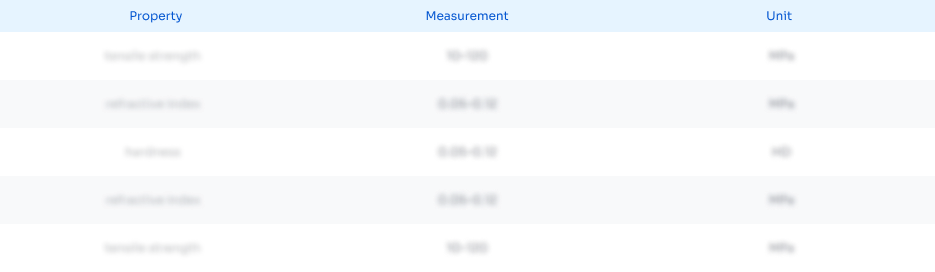
Abstract
Description
Claims
Application Information
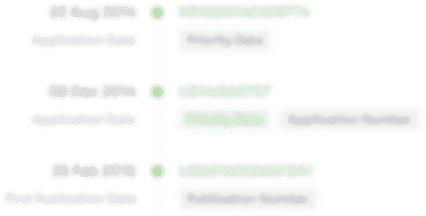
- R&D Engineer
- R&D Manager
- IP Professional
- Industry Leading Data Capabilities
- Powerful AI technology
- Patent DNA Extraction
Browse by: Latest US Patents, China's latest patents, Technical Efficacy Thesaurus, Application Domain, Technology Topic, Popular Technical Reports.
© 2024 PatSnap. All rights reserved.Legal|Privacy policy|Modern Slavery Act Transparency Statement|Sitemap|About US| Contact US: help@patsnap.com