BERT-BiGRU-IDCNN-CRF named entity identification method based on attention mechanism
A technology of named entity recognition and attention, which is applied in the direction of neural learning methods, instruments, biological neural network models, etc., can solve problems such as labor-intensive, error-prone, and corpus-dependent, and achieve improved accuracy, fast training speed, and training The effect of fewer parameters
- Summary
- Abstract
- Description
- Claims
- Application Information
AI Technical Summary
Problems solved by technology
Method used
Image
Examples
Embodiment Construction
[0070] The technical solutions in the embodiments of the present invention will be described clearly and in detail below with reference to the drawings in the embodiments of the present invention. The described embodiments are only some of the embodiments of the invention.
[0071] The technical scheme that the present invention solves the problems of the technologies described above is:
[0072] Such as figure 1 As shown, it is a schematic flow chart of the BERT-BiGRU-IDCNN-CRF named entity recognition method based on the attention mechanism of the present invention. Include the following steps:
[0073] S1. Training the BERT model based on large-scale unlabeled predictions;
[0074] Specifically, the structure of the BERT model is as follows figure 2 As shown, it mainly includes the model embedding layer, bidirectional Transformer encoding and output layer.
[0075] The embedding layer is the input of the model, which is the sum of word embedding, position embedding, a...
PUM
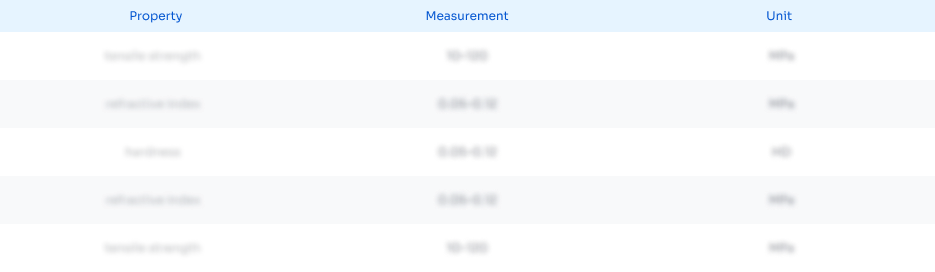
Abstract
Description
Claims
Application Information
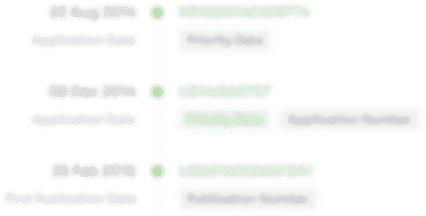
- Generate Ideas
- Intellectual Property
- Life Sciences
- Materials
- Tech Scout
- Unparalleled Data Quality
- Higher Quality Content
- 60% Fewer Hallucinations
Browse by: Latest US Patents, China's latest patents, Technical Efficacy Thesaurus, Application Domain, Technology Topic, Popular Technical Reports.
© 2025 PatSnap. All rights reserved.Legal|Privacy policy|Modern Slavery Act Transparency Statement|Sitemap|About US| Contact US: help@patsnap.com