A neural network-assisted method for the synthesis of chemical materials
A neural network and chemical technology, applied in the field of new material research and development, can solve the problems of dimensionality reduction, ambiguity, incompatibility, etc., and achieve the effect of improving the success rate, good adaptability and saving time.
- Summary
- Abstract
- Description
- Claims
- Application Information
AI Technical Summary
Problems solved by technology
Method used
Image
Examples
Embodiment
[0034] like figure 1 As shown, the method of neural network-assisted chemical material synthesis includes the following steps:
[0035] Step 1: Obtain 3955 complete chemical reaction data from CSD and ZINC databases, remove useless attributes and non-numeric fields, and perform one-hot encoding;
[0036] Each chemical reaction data includes chemical molecule name, chemical molecule physical and chemical properties, atomic properties, reaction conditions (temperature, reaction time, pH value, etc.), reactant molar ratio, reaction product crystallization data, etc. 293 dimensions of attribute characteristics, Remove non-numeric fields and attribute columns that cannot describe their own distribution rules (such as Co attribute value, 99.9% of the attribute values are -1, and only 0.1% of the value is 1), and label the products of each chemical reaction. The outcome value is 1, otherwise it is 0;
[0037] Step 2, use formula (1) to calculate the correlation coefficient ρ of a...
PUM
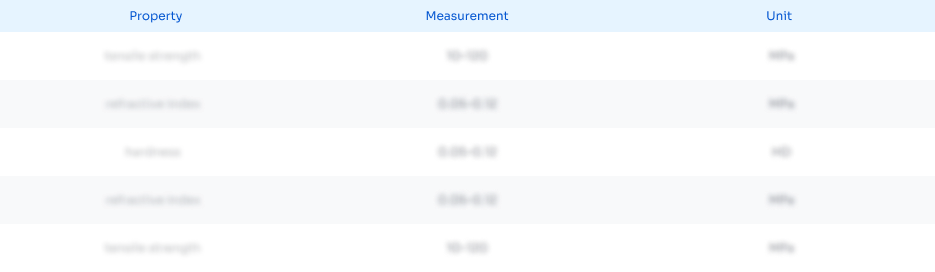
Abstract
Description
Claims
Application Information
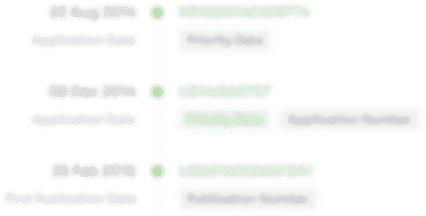
- R&D Engineer
- R&D Manager
- IP Professional
- Industry Leading Data Capabilities
- Powerful AI technology
- Patent DNA Extraction
Browse by: Latest US Patents, China's latest patents, Technical Efficacy Thesaurus, Application Domain, Technology Topic, Popular Technical Reports.
© 2024 PatSnap. All rights reserved.Legal|Privacy policy|Modern Slavery Act Transparency Statement|Sitemap|About US| Contact US: help@patsnap.com