Method for detecting X-ray mammary gland lesion image based on feature pyramid network under transfer learning
A feature pyramid, transfer learning technology, applied in neural learning methods, image enhancement, image analysis and other directions, can solve the problem that parameters cannot be optimized, the mathematical model of cross entropy loss function and related parameters are not given, and the error is large.
- Summary
- Abstract
- Description
- Claims
- Application Information
AI Technical Summary
Problems solved by technology
Method used
Image
Examples
Embodiment Construction
[0065] The present invention will be further described below in conjunction with the drawings and specific embodiments, but the embodiments described by the drawings are exemplary and are only used to explain the present invention and cannot limit the scope of the present invention.
[0066] Such as figure 1 As shown, this embodiment provides a feature pyramid network under transfer learning to mammography X-ray (X-ray) breast lesion image detection method, and its specific implementation steps are as follows:
[0067] Step 1. Establish source domain and target domain datasets: the small-sample image dataset Data_A is used as the target domain data, and the large-scale image dataset Data_B is used as the source domain data;
[0068] In this step, establish or obtain a large number of open-source CT chest image datasets, standardize them and form the source dataset Data_B, use Data_B to train the upper branch model parameters, and the source domain detection results are only us...
PUM
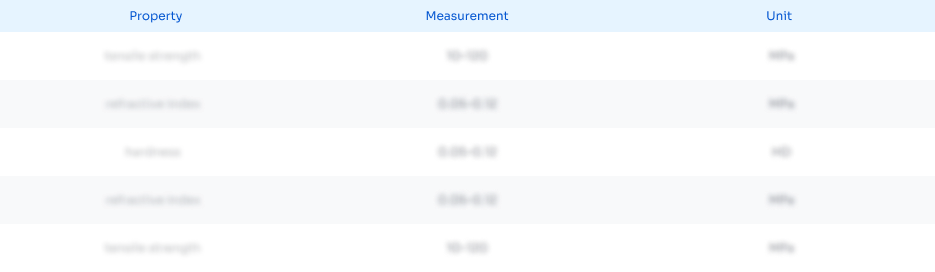
Abstract
Description
Claims
Application Information
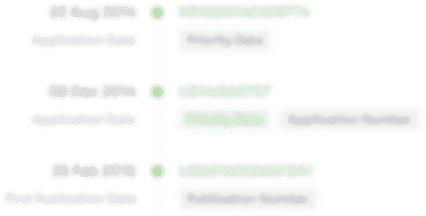
- R&D Engineer
- R&D Manager
- IP Professional
- Industry Leading Data Capabilities
- Powerful AI technology
- Patent DNA Extraction
Browse by: Latest US Patents, China's latest patents, Technical Efficacy Thesaurus, Application Domain, Technology Topic, Popular Technical Reports.
© 2024 PatSnap. All rights reserved.Legal|Privacy policy|Modern Slavery Act Transparency Statement|Sitemap|About US| Contact US: help@patsnap.com