Data generation method and device, equipment and storage medium
A data generation and data technology, applied in the field of machine learning, can solve the problem that training samples are not easy to obtain in large quantities, and achieve the effect of improving the accuracy of the generative model
- Summary
- Abstract
- Description
- Claims
- Application Information
AI Technical Summary
Problems solved by technology
Method used
Image
Examples
Embodiment 1
[0058] figure 1 It is a flow chart of a data generation method provided by Embodiment 1 of the present invention. This embodiment is applicable to the situation of generating dummy data. The method can be executed by a data generation device, and the device can be implemented by software and / or hardware.
[0059] Such as figure 1 As shown, the method specifically includes the following steps:
[0060] Step 110. Determine the Voronoi weights of each first data in the first data set in the space convex region.
[0061] In this embodiment, the first data set and the second data set are two data sets for calculating the target mapping relationship, the first data set includes a plurality of first data, and the second data set includes a plurality of second data.
[0062] Specifically, the first data set containing the first data can be obtained, and in the minimum spatial convex region surrounding the first data set, a Voronoi diagram of the first data set in the spatial convex ...
Embodiment 2
[0071] image 3 It is a flow chart of a data generation method provided by Embodiment 2 of the present invention. In this embodiment, on the basis of the foregoing embodiments, the foregoing data generation method is further optimized.
[0072] Such as image 3 As shown, the method specifically includes:
[0073] Step 210: Obtain the first data set including the first data, and determine the smallest convex area including all the first data as the spatial convex area.
[0074] In this embodiment, after the first data set is acquired, the minimum d-dimensional spatial convex area surrounding all the first data can be recorded as a hypercube C, where d is the dimension of the first data in the first data set.
[0075] Step 220, based on the data distribution of each first data, construct a Voronoi diagram of the spatial convex region.
[0076] Wherein, the Voronoi cells in the Voronoi diagram are in one-to-one correspondence with the first data in the first data set, and eac...
Embodiment 3
[0115] The data generation device provided by the embodiment of the present invention can execute the data generation method provided by any embodiment of the present invention, and has corresponding functional modules and beneficial effects for executing the method. image 3 It is a structural block diagram of a data generation device provided in Embodiment 3 of the present invention, such as image 3 As shown, the device includes: a Voronoi weight determination module 310 , a target mapping relationship determination module 320 and a data generation module 330 .
[0116] The Voronoi weight determination module 310 is configured to determine the Voronoi weights of each first data in the first data set in a spatially convex region.
[0117] The target mapping relationship determination module 320 is configured to adjust the initial mapping relationship between the first data set and the second data set according to the Voronoi weights of each of the first data to obtain a targ...
PUM
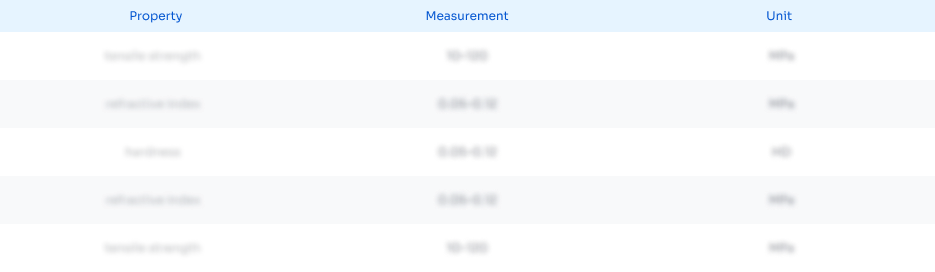
Abstract
Description
Claims
Application Information
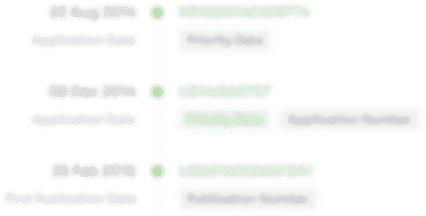
- R&D
- Intellectual Property
- Life Sciences
- Materials
- Tech Scout
- Unparalleled Data Quality
- Higher Quality Content
- 60% Fewer Hallucinations
Browse by: Latest US Patents, China's latest patents, Technical Efficacy Thesaurus, Application Domain, Technology Topic, Popular Technical Reports.
© 2025 PatSnap. All rights reserved.Legal|Privacy policy|Modern Slavery Act Transparency Statement|Sitemap|About US| Contact US: help@patsnap.com