Adversarial sample attack-resistant speech recognition model training method
A speech recognition model and adversarial sample technology, applied in speech recognition, speech analysis, instruments, etc., can solve problems such as the inability of the human ear to recognize disturbances, and neural network adversarial attacks.
- Summary
- Abstract
- Description
- Claims
- Application Information
AI Technical Summary
Problems solved by technology
Method used
Image
Examples
Embodiment Construction
[0046] Below in conjunction with accompanying drawing and embodiment the present invention is described in detail:
[0047] like figure 1 As shown, the speech recognition model training method against adversarial sample attack of the present invention comprises the following steps:
[0048] A: Select a data set consisting of N audio files, and record the Chinese character corresponding to the audio file as y;
[0049] In the present invention, the Chinese speech recognition framework and the Chinese speech data set Free ST-Chinese-Mandarin-Corpus are adopted. The Free ST-Chinese-Mandarin-Corpus dataset consists of N audio files. Each audio file is a sentence read by a reader. Each sentence contains about ten Chinese characters. The corresponding audio files Chinese characters are recorded as y.
[0050] B: Sampling the voice files in the data set selected in step A to obtain the collection tensor;
[0051] When preprocessing the voice file, first cut off the invalid part o...
PUM
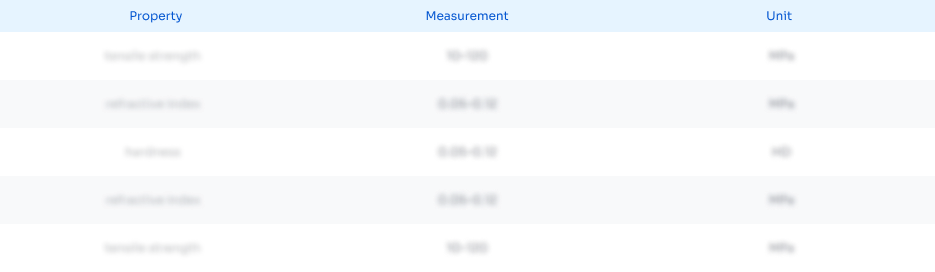
Abstract
Description
Claims
Application Information
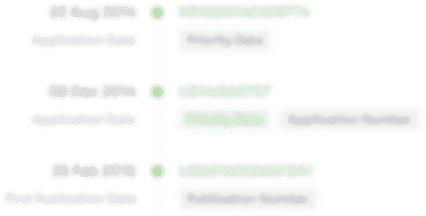
- R&D
- Intellectual Property
- Life Sciences
- Materials
- Tech Scout
- Unparalleled Data Quality
- Higher Quality Content
- 60% Fewer Hallucinations
Browse by: Latest US Patents, China's latest patents, Technical Efficacy Thesaurus, Application Domain, Technology Topic, Popular Technical Reports.
© 2025 PatSnap. All rights reserved.Legal|Privacy policy|Modern Slavery Act Transparency Statement|Sitemap|About US| Contact US: help@patsnap.com