Unsupervised semantic segmentation method for cross-domain remote sensing image
A remote sensing image and semantic segmentation technology, applied in image analysis, image enhancement, image data processing, etc., can solve the problem of low segmentation accuracy, achieve high segmentation accuracy, improve segmentation performance, and improve generalization performance.
- Summary
- Abstract
- Description
- Claims
- Application Information
AI Technical Summary
Problems solved by technology
Method used
Image
Examples
Embodiment Construction
[0038] Exemplary embodiments of the present disclosure will be described in more detail below with reference to the accompanying drawings. Although exemplary embodiments of the present disclosure are shown in the drawings, it should be understood that the present disclosure may be embodied in various forms and should not be limited by the embodiments set forth herein. Rather, these embodiments are provided for more thorough understanding of the present disclosure and to fully convey the scope of the present disclosure to those skilled in the art.
[0039] The present invention provides an unsupervised semantic segmentation method for cross-domain remote sensing images, such as figure 1 As shown, wherein, the method includes:
[0040] S1. Obtain the unlabeled target domain remote sensing image to be segmented;
[0041] S2. Input the unlabeled target domain remote sensing image to be segmented into the pre-trained unsupervised semantic segmentation model; the unsupervised sema...
PUM
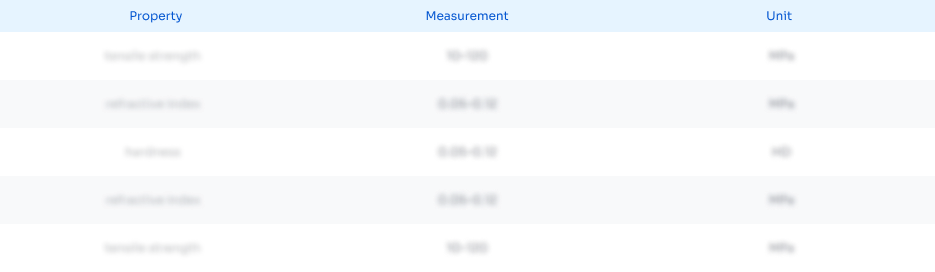
Abstract
Description
Claims
Application Information
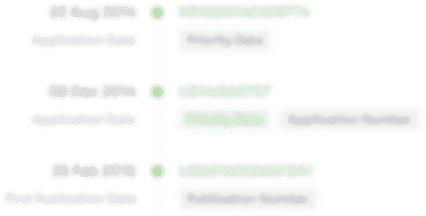
- R&D Engineer
- R&D Manager
- IP Professional
- Industry Leading Data Capabilities
- Powerful AI technology
- Patent DNA Extraction
Browse by: Latest US Patents, China's latest patents, Technical Efficacy Thesaurus, Application Domain, Technology Topic, Popular Technical Reports.
© 2024 PatSnap. All rights reserved.Legal|Privacy policy|Modern Slavery Act Transparency Statement|Sitemap|About US| Contact US: help@patsnap.com