A Behavior Recognition Method Based on Transformation Module
A technology for converting modules and recognition methods, which is applied in character and pattern recognition, neural learning methods, biological neural network models, etc., and can solve problems such as long model training period, increase in 3D convolution model parameters, and excessive model parameters. Achieve the effects of improving parallel computing capabilities, solving weak compatibility, and improving migration and deployment performance
- Summary
- Abstract
- Description
- Claims
- Application Information
AI Technical Summary
Problems solved by technology
Method used
Image
Examples
Embodiment Construction
[0125] The specific embodiment of the present invention will be further described below in conjunction with accompanying drawing and specific embodiment:
[0126] combine Figure 1-3 , a behavior recognition method based on a transformation module, comprising the following steps:
[0127] Step 1, reading continuous frame images and constructing masks, reading continuous frame images and constructing masks includes the following process:
[0128] In chronological order, continuous clip = 16 frames of image data, construct the input data input, and the continuous frame image data input has a dimension of input∈R 16×3×H×W The four-dimensional matrix of , where H, W represent the original height and width of the picture;
[0129] For each picture of the continuous frame input data input, the proportional scaling method is used to transform the size of the picture. After the above operations, the obtained data dimension is shown in formula (1):
[0130] input∈R 16×3×h×w (1)
...
PUM
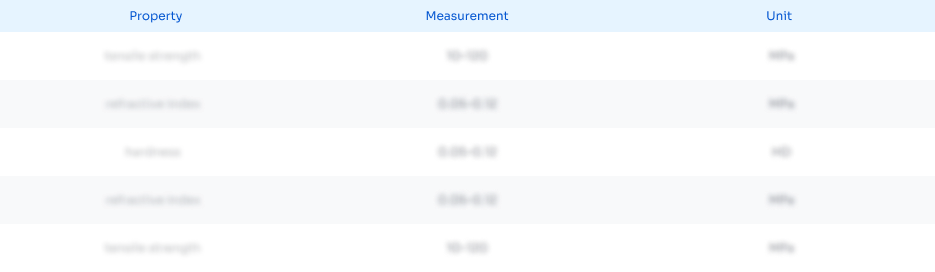
Abstract
Description
Claims
Application Information
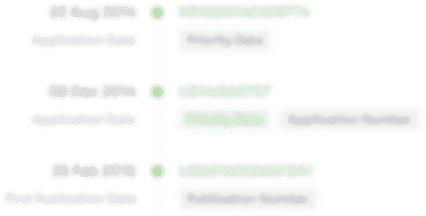
- R&D
- Intellectual Property
- Life Sciences
- Materials
- Tech Scout
- Unparalleled Data Quality
- Higher Quality Content
- 60% Fewer Hallucinations
Browse by: Latest US Patents, China's latest patents, Technical Efficacy Thesaurus, Application Domain, Technology Topic, Popular Technical Reports.
© 2025 PatSnap. All rights reserved.Legal|Privacy policy|Modern Slavery Act Transparency Statement|Sitemap|About US| Contact US: help@patsnap.com