Online car-hailing demand prediction method based on convolutional network and non-local network
A demand forecasting and convolutional network technology, applied in the field of deep learning, can solve the problems of gradient disappearance, gradient explosion, poor interpretability, etc., to avoid gradient disappearance, improve prediction accuracy, and improve computing efficiency.
- Summary
- Abstract
- Description
- Claims
- Application Information
AI Technical Summary
Problems solved by technology
Method used
Image
Examples
Embodiment Construction
[0030] The present invention will be further described below in conjunction with the accompanying drawings and embodiments.
[0031] The present invention extracts the local feature space and the global space feature from the online car-hailing demand tensor corresponding to the previous moment of the current moment, the same moment of the previous day, and the same moment of the previous week, and combines weather and time data to construct a space-time model, and then Forecast the future demand for online car-hailing.
[0032] like figure 1 and figure 2 As shown, a network-based car-hailing demand prediction method based on convolutional network and non-local network includes the following steps:
[0033] Step 1: Data processing;
[0034] Step 1-1: First obtain the car-hailing data and weather data of historical online car-hailing users in the last 6 months, and divide the city into equal-grained grids according to the given spatial granularity to obtain multiple urban a...
PUM
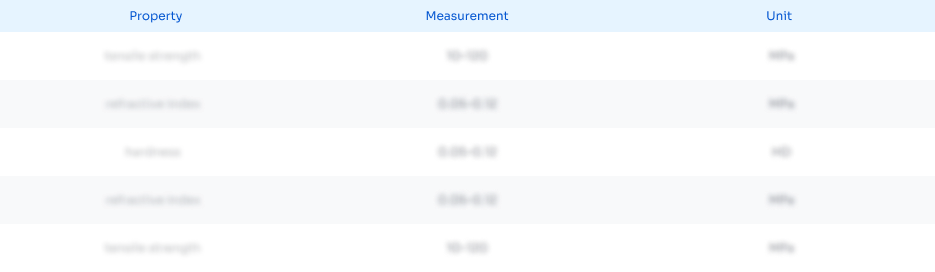
Abstract
Description
Claims
Application Information
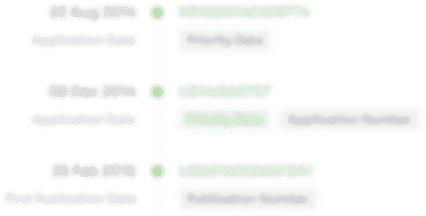
- R&D Engineer
- R&D Manager
- IP Professional
- Industry Leading Data Capabilities
- Powerful AI technology
- Patent DNA Extraction
Browse by: Latest US Patents, China's latest patents, Technical Efficacy Thesaurus, Application Domain, Technology Topic, Popular Technical Reports.
© 2024 PatSnap. All rights reserved.Legal|Privacy policy|Modern Slavery Act Transparency Statement|Sitemap|About US| Contact US: help@patsnap.com