A 3D point cloud reconstruction method based on deep learning
A 3D point cloud and deep learning technology, applied in the field of 3D point cloud reconstruction based on deep learning, can solve problems such as rough 3D point cloud and sparseness, and achieve the effect of alleviating cross-view differences
- Summary
- Abstract
- Description
- Claims
- Application Information
AI Technical Summary
Problems solved by technology
Method used
Image
Examples
Embodiment Construction
[0032] In order to make the objectives, technical solutions and advantages of the present invention clearer, the embodiments of the present invention are further described in detail below.
[0033] An embodiment of the present invention provides a deep learning-based three-dimensional point cloud reconstruction method, see figure 1 , the method includes the following steps:
[0034] 1. Build a sparse point cloud reconstruction module
[0035] First, a sparse point cloud reconstruction module is constructed, which consists of multiple identical sparse point cloud reconstruction subnetworks. Each sparse point cloud reconstruction subnet includes: a feature encoder and a point cloud predictor.
[0036] (1) Feature encoder: a deep learning-based two-dimensional image feature extraction network VGG16 is used. The input of the VGG16 network is a picture taken or projected from a three-dimensional object from a certain perspective. The network is used to learn visual information f...
PUM
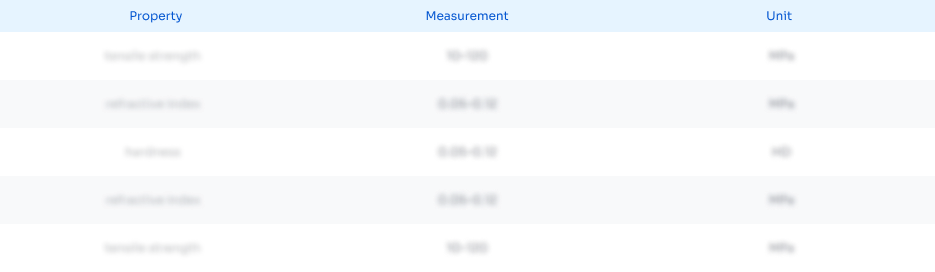
Abstract
Description
Claims
Application Information
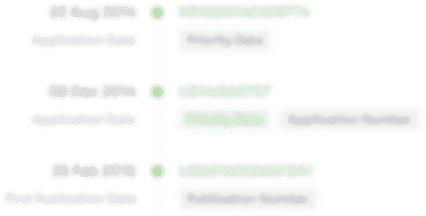
- R&D
- Intellectual Property
- Life Sciences
- Materials
- Tech Scout
- Unparalleled Data Quality
- Higher Quality Content
- 60% Fewer Hallucinations
Browse by: Latest US Patents, China's latest patents, Technical Efficacy Thesaurus, Application Domain, Technology Topic, Popular Technical Reports.
© 2025 PatSnap. All rights reserved.Legal|Privacy policy|Modern Slavery Act Transparency Statement|Sitemap|About US| Contact US: help@patsnap.com