Data processing module, data processing system and data processing method
A technology for processing modules and processing facilities, which is applied in the field of mapping of elements and their synapses, and can solve problems such as increased power consumption and waste of resources
- Summary
- Abstract
- Description
- Claims
- Application Information
AI Technical Summary
Problems solved by technology
Method used
Image
Examples
Embodiment approach
[0069] The input synapse ID is represented by the address index of the memory cell itself (no memory bits are used for this information). Each addressable entry in this memory unit corresponds to a specified synapse. The depth of the storage unit is a1.
[0070] In the example shown, the field Neural Unit ID includes an identifier for the neuron. The required size b1 of this field is 2log of the number of neurons (eg for a data processing module with 256 neurons this field would be 8 bits).
[0071] The second field contains a value representing the synapse weight assigned to the synapse. The number of bits b2 of this field can be smaller or larger depending on the desired granularity used to specify synaptic weights. In the example, the number of bits of this field is 32 bits.
[0072] Mapping example
[0073] The table below shows the image 3 The filling content of this storage unit 14 of the exemplary network is shown. The exemplary network has three neurons (N0, ...
example 3
[0119] As another example, the contents of memories 12, 13, and 14 are described for unsigned memories, as Figure 2D shown.
[0120] Table 11: Exemplary Input Synaptic Storage Units
[0121] Enter Synapse ID neuron ID synaptic weight D0 N1 we D1 N2 0.5we D2 N1 wi D3 N3 wacc D4 N3 -wacc D5 N5 we D6 N3 wacc D7 N5 we
[0122] Table 12: Exemplary Output Synaptic Storage Units
[0123] output synapse ID synaptic delay Destination ID Enter Synapse ID A0 Tsyn NEX D0 A1 Tsyn NEX D1 A2 Tsyn NEX D2 A3 Tsyn+Tmin NEX D3 A4 Tsyn NEX D4 A5 Tsyn NEX D5 A6 Tsyn NEX D6 A7 2*Tsyn+Tneu NEX D7 - - - - - - - -
[0124] Table 13: Output Synaptic Slice Storage Units
[0125] neuron ID Offset number of output synapses N0 0 2 N1 2 2 N2 4 1 N3 5 1 N4 6 2 N5 - - ...
PUM
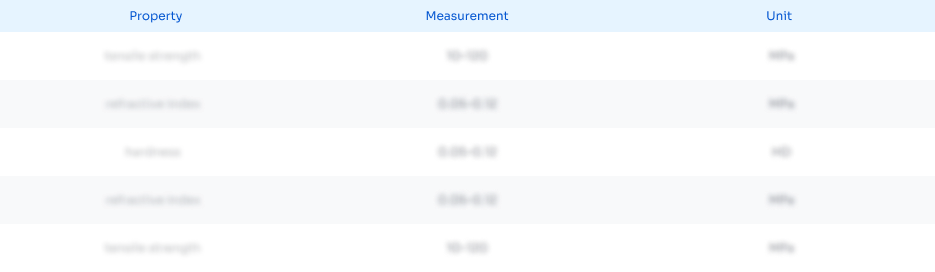
Abstract
Description
Claims
Application Information
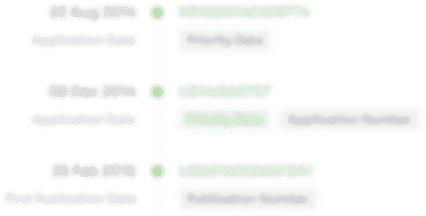
- R&D
- Intellectual Property
- Life Sciences
- Materials
- Tech Scout
- Unparalleled Data Quality
- Higher Quality Content
- 60% Fewer Hallucinations
Browse by: Latest US Patents, China's latest patents, Technical Efficacy Thesaurus, Application Domain, Technology Topic, Popular Technical Reports.
© 2025 PatSnap. All rights reserved.Legal|Privacy policy|Modern Slavery Act Transparency Statement|Sitemap|About US| Contact US: help@patsnap.com