Video and text cross-modal retrieval method based on relational reasoning network
A cross-modal and relational technology, applied in the field of cross-modal retrieval of video and text, can solve the problem of ignoring the internal information relationship of a single modality, failing to extract information in the time domain well, and the expression of single-modal information is not complete and sufficiency, etc., to achieve a good cross-modal retrieval effect
- Summary
- Abstract
- Description
- Claims
- Application Information
AI Technical Summary
Problems solved by technology
Method used
Image
Examples
Embodiment 1
[0048] This embodiment proposes a cross-modal retrieval method for video and text based on a relational reasoning network, the flow chart of which is shown in figure 1 , wherein the method includes the following steps:
[0049] Step 1. Extract video data features and text data features.
[0050] Step 2. Use the cyclic neural network to obtain the global features of the video and the global features of the text.
[0051] Step 3. Use the multi-scale relational inference network to construct video local relation features and text local relation features.
[0052] Step 4. Fusion of global features and local relational features of single-modal data to obtain video fusion features and text fusion features.
[0053] Step 5. Map video fusion features and text fusion features to the common space, and align the distribution of video fusion features and text fusion features in the common space.
[0054] Step 6. Train the overall network of steps 1-5.
[0055] Step 7. Utilize the trai...
Embodiment 2
[0057] see figure 2 and image 3 , the video and text cross-modal retrieval method based on the relational reasoning network proposed in this embodiment can extract the dependencies between different frames at multiple time scales through the relational reasoning network according to the dependencies between video frames, Construct the implicit relationship between multiple frames, obtain local relationship features, and construct global features at the same time, and fuse multi-scale local relationship features and global features to form a strong semantic semantic feature as a fusion feature of the video.
[0058]In addition, according to the dependency relationship between text words, through the relationship reasoning network, the dependency relationship between different words is extracted at multiple scales, the implicit relationship between multiple words is constructed, local relationship features are obtained, and global features are constructed at the same time. An...
Embodiment 3
[0063] see Figure 4 , the cross-modal retrieval method of video and text based on the relational reasoning network proposed in this embodiment first constructs the model for training, then trains the entire network, and then performs cross-modal retrieval, mainly including steps S1-step S6 .
[0064] Step S1: Extract multimodal data features.
[0065] Multimodal data includes video, text, etc. These raw data are expressed in a way that humans can accept, but computers cannot directly process them. Their features need to be extracted and expressed in numbers that computers can process.
[0066] Wherein, step S1 specifically includes the following steps:
[0067] Step S11: For the video, use the convolutional neural network ResNet to perform feature extraction, and the video feature sequence is expressed as , where n is the number of frame sequences;
[0068] Step S12: For text, use Glove to carry out feature extraction, text feature sequence is expressed as , where m is...
PUM
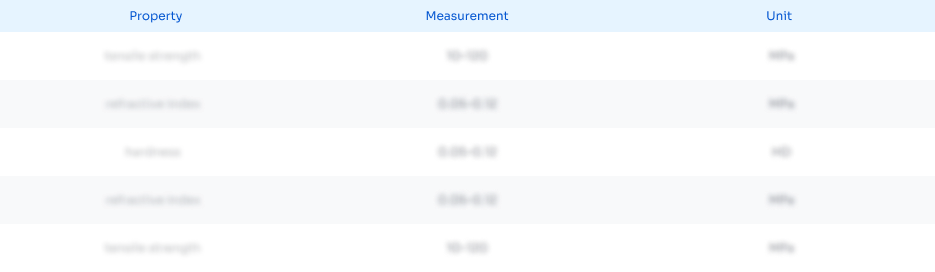
Abstract
Description
Claims
Application Information
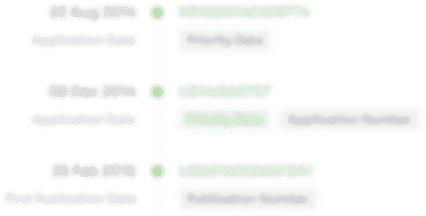
- R&D Engineer
- R&D Manager
- IP Professional
- Industry Leading Data Capabilities
- Powerful AI technology
- Patent DNA Extraction
Browse by: Latest US Patents, China's latest patents, Technical Efficacy Thesaurus, Application Domain, Technology Topic, Popular Technical Reports.
© 2024 PatSnap. All rights reserved.Legal|Privacy policy|Modern Slavery Act Transparency Statement|Sitemap|About US| Contact US: help@patsnap.com