A semantic segmentation method and system based on continuous learning
A technology of semantic segmentation and learning algorithm, applied in the field of semantic segmentation methods and systems based on continuous learning, can solve the problems of inability to obtain, forget, and consistent performance, and achieve the effect of improving average performance and alleviating the problem of catastrophic forgetting.
- Summary
- Abstract
- Description
- Claims
- Application Information
AI Technical Summary
Problems solved by technology
Method used
Image
Examples
Embodiment Construction
[0045] The following will clearly and completely describe the technical solutions in the embodiments of the present invention with reference to the accompanying drawings in the embodiments of the present invention. Obviously, the described embodiments are only some, not all, embodiments of the present invention. Based on the embodiments of the present invention, all other embodiments obtained by persons of ordinary skill in the art without creative efforts fall within the protection scope of the present invention.
[0046] Before introducing the embodiments of the present invention, the relevant terms involved in the embodiments of the present invention are first explained as follows:
[0047] RGB image: refers to the RGB image collected by the vehicle camera, which is a three-channel image.
[0048] Scene: refers to the scene generated by the environment change during the driving process of the vehicle. For example, a crowded scene when there are many vehicles around, a flas...
PUM
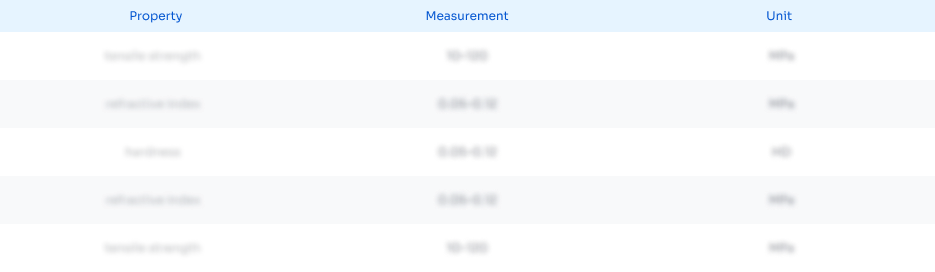
Abstract
Description
Claims
Application Information
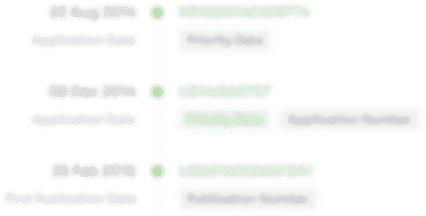
- R&D Engineer
- R&D Manager
- IP Professional
- Industry Leading Data Capabilities
- Powerful AI technology
- Patent DNA Extraction
Browse by: Latest US Patents, China's latest patents, Technical Efficacy Thesaurus, Application Domain, Technology Topic, Popular Technical Reports.
© 2024 PatSnap. All rights reserved.Legal|Privacy policy|Modern Slavery Act Transparency Statement|Sitemap|About US| Contact US: help@patsnap.com