Sequence recommendation method fusing dynamic knowledge graph
A technology of knowledge graph and recommendation method, applied in the field of sequence recommendation by integrating dynamic knowledge graph as auxiliary information
- Summary
- Abstract
- Description
- Claims
- Application Information
AI Technical Summary
Problems solved by technology
Method used
Image
Examples
Embodiment Construction
[0010] Below in conjunction with accompanying drawing and specific implementation mode, the present invention is described further, and present embodiment adopts the JDATA data set that extracts from the famous e-commerce website Jingdong of China:
[0011] Such as figure 1 As shown, the data set is obtained, and the data set is preprocessed to form an item sequence and an operation sequence, and then the session information is modeled, and the item embedding and the operation embedding are learned through the gated graph neural network and the recurrent neural network, respectively, to generate a session express. The embedding learning of the dynamic knowledge map is to learn the node characteristics through the graph convolutional neural network. The long short-term memory network updates the weight matrix at time t based on the current and historical information, and then performs the knowledge map embedding learning through TransE to obtain the loss function of the knowled...
PUM
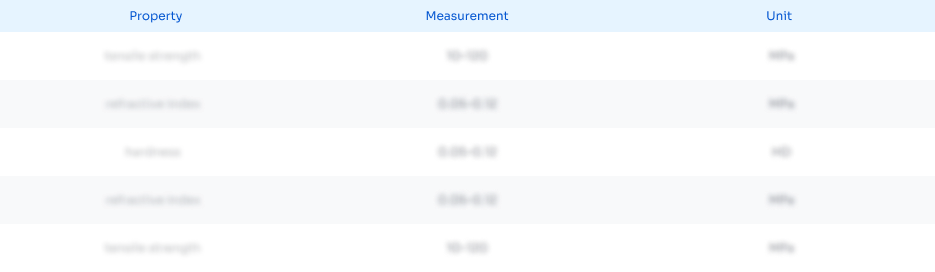
Abstract
Description
Claims
Application Information
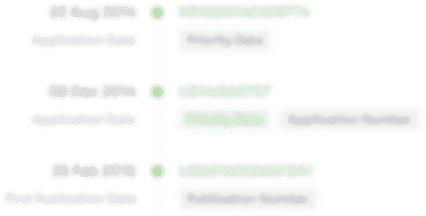
- R&D Engineer
- R&D Manager
- IP Professional
- Industry Leading Data Capabilities
- Powerful AI technology
- Patent DNA Extraction
Browse by: Latest US Patents, China's latest patents, Technical Efficacy Thesaurus, Application Domain, Technology Topic, Popular Technical Reports.
© 2024 PatSnap. All rights reserved.Legal|Privacy policy|Modern Slavery Act Transparency Statement|Sitemap|About US| Contact US: help@patsnap.com