Day-ahead electricity price prediction method based on EEMD-CNN + SAE-RFR hybrid algorithm
A technology that combines algorithms and forecasting methods, applied in market forecasting, neural learning methods, computing and other directions, can solve the problems of low forecasting accuracy, slow learning and training convergence, limited statistical model capabilities, etc., to improve stability and effectiveness, speed up Convergence speed, the effect of avoiding modal aliasing
- Summary
- Abstract
- Description
- Claims
- Application Information
AI Technical Summary
Problems solved by technology
Method used
Image
Examples
Embodiment Construction
[0057] The present invention will be further described below in conjunction with the accompanying drawings and specific embodiments, so that those skilled in the art can better understand the present invention and implement it, but the examples given are not intended to limit the present invention.
[0058] The day-ahead electricity price prediction method based on EEMD-CNN+SAE-RFR hybrid algorithm in the preferred embodiment of the present invention comprises the following steps:
[0059] A. Combining the original data of historical electricity prices and their influencing factors to form the original characteristic time series matrix of the current forecast day d.
[0060] Specific price-related factors such as figure 1 As shown, the input data structure of the deep learning network model is the time series of historical electricity prices and its influencing factors, which include the historical electricity prices of the day a before the forecast date, the power demand / load...
PUM
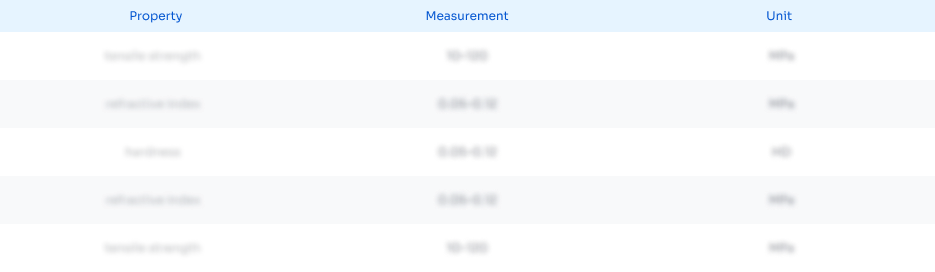
Abstract
Description
Claims
Application Information
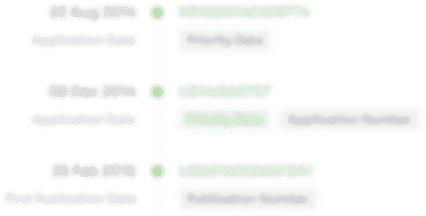
- R&D Engineer
- R&D Manager
- IP Professional
- Industry Leading Data Capabilities
- Powerful AI technology
- Patent DNA Extraction
Browse by: Latest US Patents, China's latest patents, Technical Efficacy Thesaurus, Application Domain, Technology Topic, Popular Technical Reports.
© 2024 PatSnap. All rights reserved.Legal|Privacy policy|Modern Slavery Act Transparency Statement|Sitemap|About US| Contact US: help@patsnap.com