Face recognition method based on cosine loss function
A loss function, face recognition technology, applied in the field of face recognition, can solve problems such as low training efficiency and inability to apply face recognition training, and achieve the effect of improving precision and accuracy and improving efficiency
- Summary
- Abstract
- Description
- Claims
- Application Information
AI Technical Summary
Problems solved by technology
Method used
Image
Examples
Embodiment 2
[0041] In this embodiment, a further improvement is made on the basis of the method provided in Embodiment 1. The cosine loss function designed in Embodiment 1 can make the angle between the face vector codes corresponding to the same person as small as possible, and make the face vector codes of different faces angle as large as possible.
[0042] Therefore, in this implementation, during the training process, the Embedding output by the CNN automatically matches the eigenvector encoding solved by the Thomson Problem.
[0043] Specifically, in step 4, using the cosine loss function to perform the nearest matching calculation process on the first face feature code and the second face feature code includes: respectively inputting the first face feature code and the second face feature code into the designed cosine In the loss function, the included angle θ1 of the first face feature code and the included angle θ2 of the second face feature code are calculated respectively; acco...
Embodiment 3
[0049] In the present embodiment, a kind of face recognition method based on cosine loss function comprises the following steps:
[0050] Step 1: Preprocessing the face image data that needs face recognition training to form a training sample set;
[0051] Step 2: Construct a CNN network, input the training sample set into the CNN network for face recognition convolutional neural network training, and output the first face feature code after the training is completed;
[0052] Step 3: Construct the Thomson Problem model, input the training sample set into the Thomson Problem model for solving, and calculate the second facial feature code;
[0053] Step 4: Design the cosine loss function, and use the cosine loss function to perform the nearest matching calculation on the first face feature encoding and the second face feature encoding, and the calculated nearest matching result is used to correct the parameters of the CNN network model until the calculated When the latest matc...
PUM
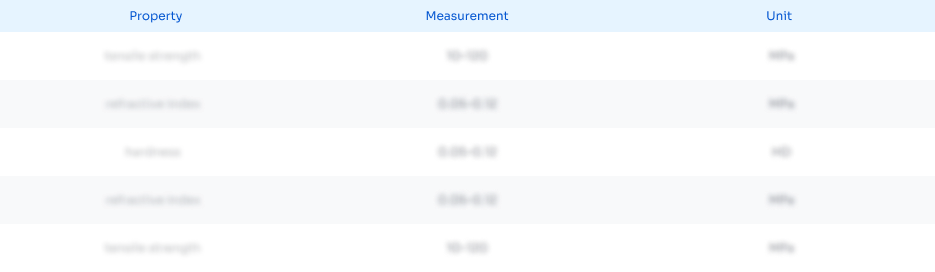
Abstract
Description
Claims
Application Information
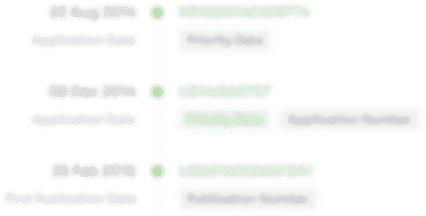
- Generate Ideas
- Intellectual Property
- Life Sciences
- Materials
- Tech Scout
- Unparalleled Data Quality
- Higher Quality Content
- 60% Fewer Hallucinations
Browse by: Latest US Patents, China's latest patents, Technical Efficacy Thesaurus, Application Domain, Technology Topic, Popular Technical Reports.
© 2025 PatSnap. All rights reserved.Legal|Privacy policy|Modern Slavery Act Transparency Statement|Sitemap|About US| Contact US: help@patsnap.com