Dynamic concept learning and knowledge graph generation method based on partial sequence three-branch structure
A technology of knowledge graph and concept learning, which is applied in the field of dynamic concept learning and knowledge graph generation based on partial order three-branch structure, which can solve problems such as time consumption, inconformity with dynamics, massive data knowledge processing requirements, knowledge graph generation, etc., to achieve The effect of efficient acquisition
- Summary
- Abstract
- Description
- Claims
- Application Information
AI Technical Summary
Problems solved by technology
Method used
Image
Examples
Embodiment
[0057] Such as figure 1 As shown, in the present invention, a dynamic concept learning and knowledge map generation method based on a partial order three-branch structure includes the following steps:
[0058] S1. For a given original form background K={U, M, I} and incremental form background K I ={U I ,M,I I} According to the binary relationship between the newly added object Δu and the original object u∈U, the category of the newly added object Δu is judged; the category of the object Δu specifically includes:
[0059] Based on the cognitive operator f, f(Δu) represents the attributes of the newly added object Δu, and Δu is divided into five categories:
[0060] The largest shared object, if f(Δu)=M, then Δu is the largest shared object;
[0061] mutually exclusive objects, for u∈U, if and and Then Δu and u are mutually exclusive objects
[0062] Nearest companion object, for u∈U, if Then Δu is a companion object of u; if Δu is a companion object of u, and u ...
PUM
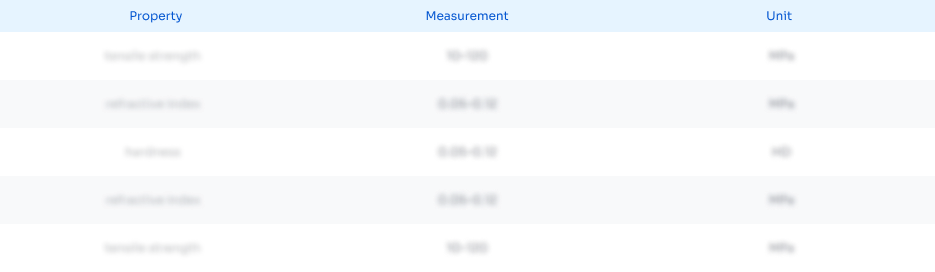
Abstract
Description
Claims
Application Information
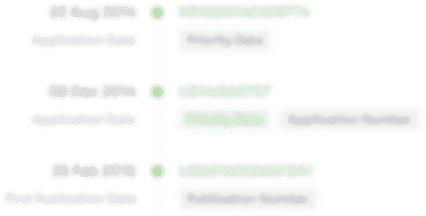
- R&D Engineer
- R&D Manager
- IP Professional
- Industry Leading Data Capabilities
- Powerful AI technology
- Patent DNA Extraction
Browse by: Latest US Patents, China's latest patents, Technical Efficacy Thesaurus, Application Domain, Technology Topic.
© 2024 PatSnap. All rights reserved.Legal|Privacy policy|Modern Slavery Act Transparency Statement|Sitemap