Knowledge distillation-based unsupervised industrial image anomaly detection method and system
An anomaly detection and image anomaly technology, applied in image analysis, image enhancement, graphics and image conversion, etc., can solve unsupervised problems, achieve the effect of improving performance and improving automation level
- Summary
- Abstract
- Description
- Claims
- Application Information
AI Technical Summary
Problems solved by technology
Method used
Image
Examples
Embodiment Construction
[0057] In order to make the object, technical solution and advantages of the present invention clearer, the present invention will be further described in detail below in conjunction with the accompanying drawings and embodiments. It should be understood that the specific embodiments described here are only used to explain the present invention, not to limit the present invention. In addition, the technical features involved in the various embodiments of the present invention described below can be combined with each other as long as they do not constitute a conflict with each other.
[0058] The present invention proposes an unsupervised industrial image anomaly detection method based on knowledge distillation, such as figure 1 shown, including:
[0059] Training phase:
[0060] Taking a normal industrial product image as a training sample image, using the sample image to train and generate an anomaly detection model, the anomaly detection model includes a teacher network a...
PUM
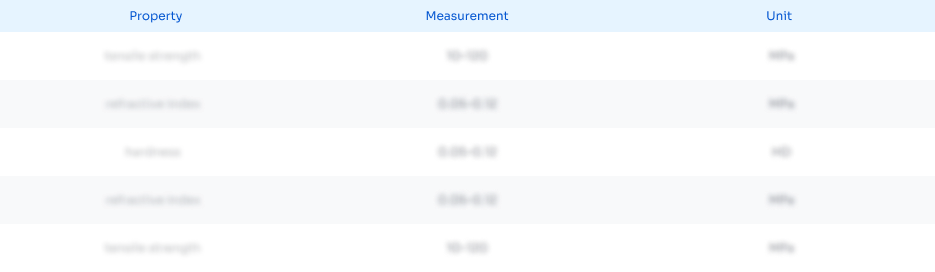
Abstract
Description
Claims
Application Information
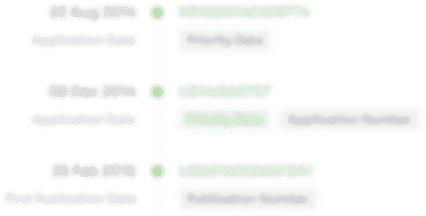
- R&D Engineer
- R&D Manager
- IP Professional
- Industry Leading Data Capabilities
- Powerful AI technology
- Patent DNA Extraction
Browse by: Latest US Patents, China's latest patents, Technical Efficacy Thesaurus, Application Domain, Technology Topic, Popular Technical Reports.
© 2024 PatSnap. All rights reserved.Legal|Privacy policy|Modern Slavery Act Transparency Statement|Sitemap|About US| Contact US: help@patsnap.com