Pseudo label loss unsupervised adversarial domain adaptive picture classification method based on Gaussian uniform mixture model
A uniformly mixed, unsupervised technology, applied in neural learning methods, biological neural network models, character and pattern recognition, etc., can solve the problem of not being able to pseudo-label to improve the accuracy of image classification
- Summary
- Abstract
- Description
- Claims
- Application Information
AI Technical Summary
Problems solved by technology
Method used
Image
Examples
Embodiment Construction
[0069] The present invention will be further described below in conjunction with the accompanying drawings. Apparently, the described embodiments are some, but not all, embodiments of the present invention. Based on the embodiments of the present invention, all other embodiments obtained by persons of ordinary skill in the art without making creative efforts belong to the protection scope of the present invention.
[0070] The present invention provides a pseudo-label loss unsupervised adversarial domain adaptive image classification method based on a Gaussian uniform mixture model. The fundamental motivation is that there are no images available for training in the target domain to be classified. Specific steps are as follows:
[0071] Step S1, acquire the images of the source domain and the target domain respectively, wherein the source domain is a known domain, the image has a label, the target domain is a domain to be classified, and the image has no label; preprocess and...
PUM
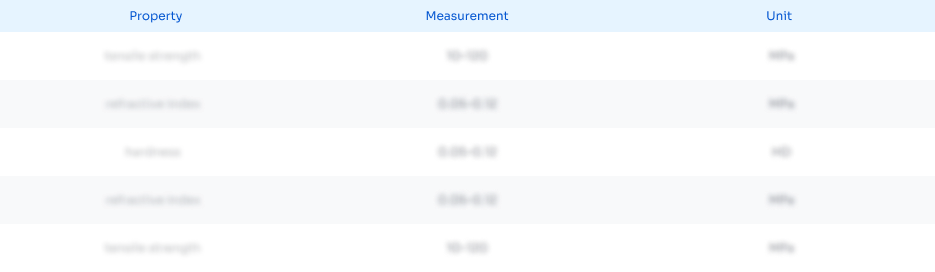
Abstract
Description
Claims
Application Information
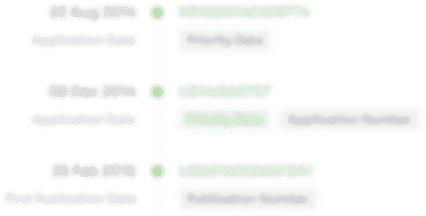
- Generate Ideas
- Intellectual Property
- Life Sciences
- Materials
- Tech Scout
- Unparalleled Data Quality
- Higher Quality Content
- 60% Fewer Hallucinations
Browse by: Latest US Patents, China's latest patents, Technical Efficacy Thesaurus, Application Domain, Technology Topic, Popular Technical Reports.
© 2025 PatSnap. All rights reserved.Legal|Privacy policy|Modern Slavery Act Transparency Statement|Sitemap|About US| Contact US: help@patsnap.com