Text clustering method based on comparative learning and integrated with dynamic adjustment mechanism
A dynamic adjustment, text clustering technology, applied in the direction of text database clustering/classification, unstructured text data retrieval, instruments, etc. Example quality, effect improvement effect
- Summary
- Abstract
- Description
- Claims
- Application Information
AI Technical Summary
Problems solved by technology
Method used
Image
Examples
Embodiment 1
[0062] see Figure 1 to Figure 2 As shown, a text clustering method based on contrastive learning and incorporating a dynamic adjustment mechanism, the specific steps of the method are as follows:
[0063] Step1. Download the public text clustering dataset from the Internet, specifically using eight datasets of SearchSnippets, StackOverflow, Biomedical, AgNews, Tweet, GoogleNews-TS, GoogleNews-T, GoogleNews-S, among which GoogleNews-TS, GoogleNews-T and GoogleNews-S are obtained by extracting titles and abstracts, respectively, from the GoogleNews [25] dataset.
[0064] The data set obtained above is shown in Table 1:
[0065] Table 1 Dataset Details
[0066]
[0067]
[0068] Step2. First, based on the context enhancement method, the enhanced text pair of the text is obtained through two different masked word prediction models, and then the feature representation is obtained by passing in the pre-trained Bert model with shared parameters, and finally the initial seman...
Embodiment 2
[0119] (1) Comparative test
[0120] Compare the effects of this experiment with other text clustering methods on eight datasets, and specifically compare the eight benchmark text clustering methods of BoW, TF-IDF, KMeans, DEC, STCC, Self-Train, HAC-SD, and SCCL. The experimental results are shown in Table 2.
[0121] Table 2 Comparison of experimental effects
[0122]
[0123]
[0124]
[0125] From the analysis of Table 2, we can see that the performance of our model exceeds the existing benchmark models on most datasets, especially compared with the SCCL model that also uses the combination of contrastive learning and clustering to achieve a certain effect.
[0126] (2) Ablation experiment
[0127] To better verify the effectiveness of our model, in this section we conduct ablation experiments. On the SearchSnippets dataset we compare the model with sequential learning, fixed-scale joint learning, and validate the effect of negative screening on the model. The ...
Embodiment 3
[0134] The present invention proposes a method for dynamically adjusting the loss weight, so as to alleviate the problem of inconsistency between comparative learning and clustering objectives. During the training process, the model adjusts the contrast loss and clustering loss by adjusting the function, so as to achieve a smooth transition from contrastive learning to clustering; by assigning pseudo-labels to the data with high confidence in the cluster assignment probability, the negative examples are screened to solve the problem. The problem that the same cluster data are negative examples of each other effectively improves the quality of negative examples. The data representation obtained by comparative learning through this method is more friendly to clustering; compared with the existing comparative clustering method, the present invention achieves a significant improvement in effect, and is superior to the existing short text clustering on most data sets method.
PUM
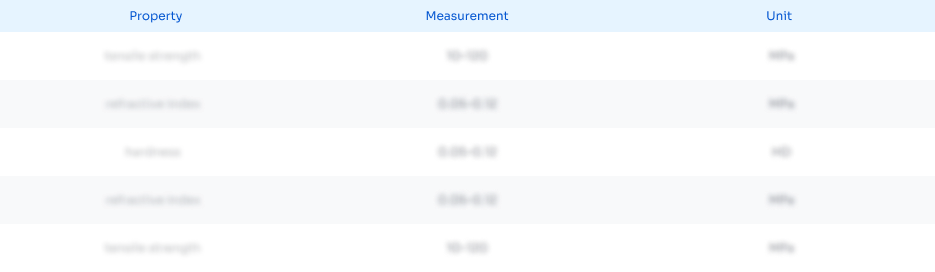
Abstract
Description
Claims
Application Information
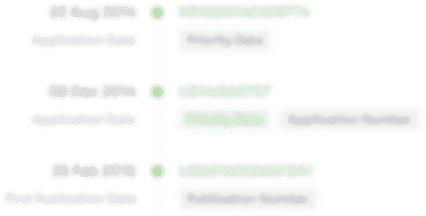
- R&D Engineer
- R&D Manager
- IP Professional
- Industry Leading Data Capabilities
- Powerful AI technology
- Patent DNA Extraction
Browse by: Latest US Patents, China's latest patents, Technical Efficacy Thesaurus, Application Domain, Technology Topic, Popular Technical Reports.
© 2024 PatSnap. All rights reserved.Legal|Privacy policy|Modern Slavery Act Transparency Statement|Sitemap|About US| Contact US: help@patsnap.com