Accelerator for end-side real-time training
An accelerator and weight technology, applied in the field of meta-learning, can solve problems such as accuracy drop and load imbalance
- Summary
- Abstract
- Description
- Claims
- Application Information
AI Technical Summary
Problems solved by technology
Method used
Image
Examples
Embodiment Construction
[0050] In order to facilitate the technical solution of the application, some concepts involved in the application are first described below.
[0051] The training of convolutional neural network generally includes three stages of calculation: FF (feed-forward, forward propagation stage), BP (backward propagation, back propagation stage) and WG (weight gradient generation, weight update stage). A batch of data is forward-propagated and their losses are obtained. The BP process obtains the error of each intermediate feature map by back-propagating the loss. The WG process uses the error of the intermediate features to obtain the gradient value and update value of the weight, and performs renew.
[0052] The specific calculations involved in the above three stages are as follows:
[0053] In the FF stage, the intermediate feature map a of the previous convolutional layer l-1 by the weight W of the current convolutional layer l convolution, and with the bias b l After the add...
PUM
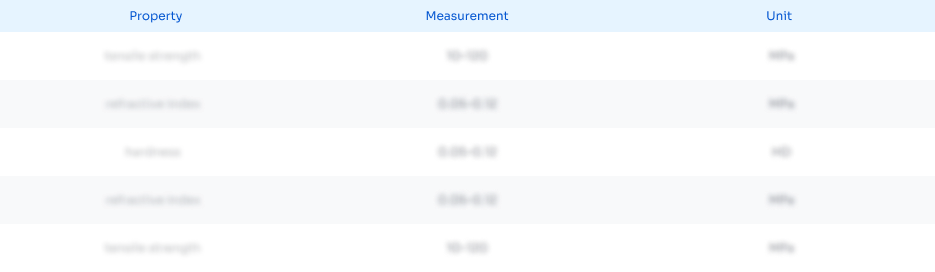
Abstract
Description
Claims
Application Information
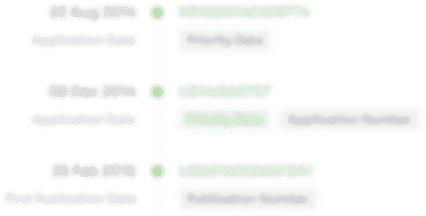
- R&D
- Intellectual Property
- Life Sciences
- Materials
- Tech Scout
- Unparalleled Data Quality
- Higher Quality Content
- 60% Fewer Hallucinations
Browse by: Latest US Patents, China's latest patents, Technical Efficacy Thesaurus, Application Domain, Technology Topic, Popular Technical Reports.
© 2025 PatSnap. All rights reserved.Legal|Privacy policy|Modern Slavery Act Transparency Statement|Sitemap|About US| Contact US: help@patsnap.com