Visual detection-oriented target detection model training method and target detection method
A technology of target detection and visual detection, which is applied in semi-supervised learning, target detection, and target detection fields, can solve the problems of consuming a lot of manpower and material resources, and achieve the effect of excellent fault tolerance and good real-time monitoring performance
- Summary
- Abstract
- Description
- Claims
- Application Information
AI Technical Summary
Problems solved by technology
Method used
Image
Examples
Embodiment Construction
[0043] The present invention will be further described in detail below with reference to the accompanying drawings. The examples are only used to explain the present invention, but not to limit the scope of the present invention.
[0044] figure 1 It is a schematic diagram of the steps of the pseudo-label training method:
[0045] (1) Use the labeled data to train the selected target detection model to obtain initial weights.
[0046] (2) Using the initial weight parameters, input unlabeled data for prediction.
[0047] (3) Obtain high-confidence annotation results as pseudo-annotations for unlabeled data.
[0048] (4) Input the labeled data and unlabeled data together into the model for retraining.
[0049] figure 2 It is a schematic diagram of consistency regularization: under the constraint of consistency regularization, the results obtained by two different forms of letter A after inputting the model prediction should be A, and the results should be consistent.
[0050...
PUM
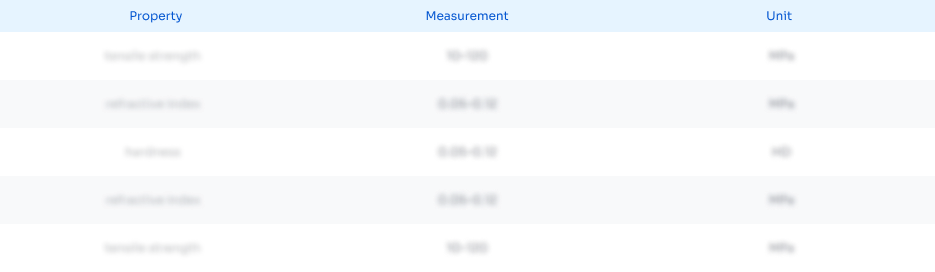
Abstract
Description
Claims
Application Information
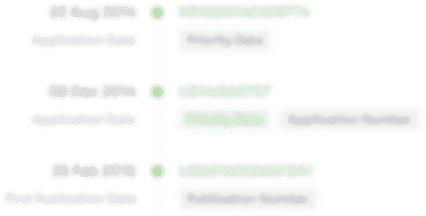
- Generate Ideas
- Intellectual Property
- Life Sciences
- Materials
- Tech Scout
- Unparalleled Data Quality
- Higher Quality Content
- 60% Fewer Hallucinations
Browse by: Latest US Patents, China's latest patents, Technical Efficacy Thesaurus, Application Domain, Technology Topic, Popular Technical Reports.
© 2025 PatSnap. All rights reserved.Legal|Privacy policy|Modern Slavery Act Transparency Statement|Sitemap|About US| Contact US: help@patsnap.com