Self-adaptive robust constraint maximum variation mapping (CMVM) feature dimension reduction method for image retrieval of plant laminae
A technology of image retrieval and plant leaves, which is applied in the fields of instruments, character and pattern recognition, computer parts, etc.
- Summary
- Abstract
- Description
- Claims
- Application Information
AI Technical Summary
Problems solved by technology
Method used
Image
Examples
Embodiment Construction
[0039] like figure 1 As shown, the adaptive robust CMVM feature dimensionality reduction method for diverse graph retrieval specifically includes the following steps:
[0040] (1) Preprocessing the plant image dataset;
[0041] (2) Using an interactive level set segmentation scheme for image segmentation;
[0042] After research, it is found that the segmentation method based on threshold is simple and easy to implement, but it has great limitations, and can only effectively deal with images with relatively simple backgrounds; for leaf images with complex backgrounds, the interaction-based Snake method and watershed method are compared Works, but requires a lot of interaction time to set up initial outlines and markers, which is less efficient. And the level set method is because it is suitable for dealing with the complex topological structure change, has stronger curve approximation ability, segmentation precision is higher and other significant features, so it is more sui...
PUM
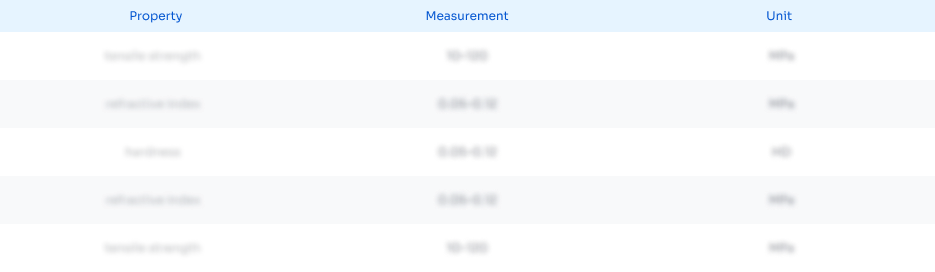
Abstract
Description
Claims
Application Information
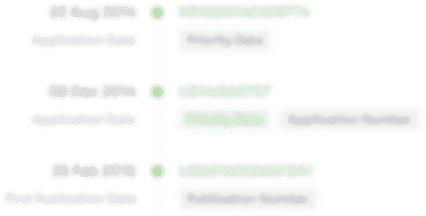
- R&D Engineer
- R&D Manager
- IP Professional
- Industry Leading Data Capabilities
- Powerful AI technology
- Patent DNA Extraction
Browse by: Latest US Patents, China's latest patents, Technical Efficacy Thesaurus, Application Domain, Technology Topic, Popular Technical Reports.
© 2024 PatSnap. All rights reserved.Legal|Privacy policy|Modern Slavery Act Transparency Statement|Sitemap|About US| Contact US: help@patsnap.com