Image classification method based on characteristic weight learning and nuclear sparse representation
A technology of kernel sparse representation and classification method, which is applied in the fields of instruments, character and pattern recognition, computer parts, etc.
- Summary
- Abstract
- Description
- Claims
- Application Information
AI Technical Summary
Problems solved by technology
Method used
Image
Examples
specific Embodiment approach
[0037] refer to figure 1 , the specific embodiment of the present invention is as follows:
[0038] Step 1, input the training set and map it to the kernel subspace
[0039] First, input the training set X'={X' 1 ,X′ 2 ,...,X' C}, where X' p Represents the collection of the pth class samples in the training set, p=1,2,...,C, C represents the number of sample categories in the training set;
[0040] Secondly, use nonlinear kernel mapping, that is, the following Gaussian kernel function k(x, y), to map the samples of the training set X′ in the input sample space to the kernel space, and obtain the training set X″=[k(x i ,x j )] n×n ,
[0041] k(x,y)=exp(-t||x-y|| 2 ),
[0042] Wherein, x and y represent any two samples, ||·|| represents the distance between x and y, exp(·) is an exponential function, and t>0 is a parameter of the Gaussian kernel, which is set as the median value of is the mean of all training samples, x i and x j are the i-th and j-th training sa...
PUM
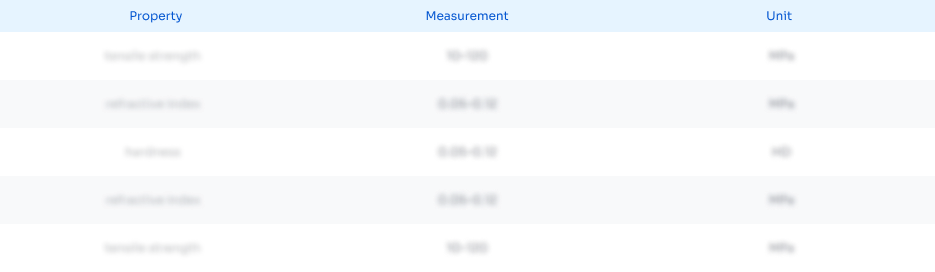
Abstract
Description
Claims
Application Information
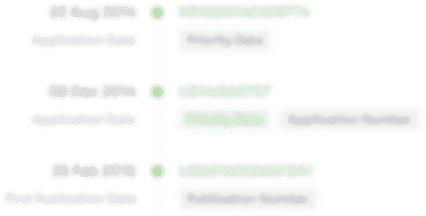
- R&D Engineer
- R&D Manager
- IP Professional
- Industry Leading Data Capabilities
- Powerful AI technology
- Patent DNA Extraction
Browse by: Latest US Patents, China's latest patents, Technical Efficacy Thesaurus, Application Domain, Technology Topic, Popular Technical Reports.
© 2024 PatSnap. All rights reserved.Legal|Privacy policy|Modern Slavery Act Transparency Statement|Sitemap|About US| Contact US: help@patsnap.com