Sparse Constrained Adaptive NLM Super-resolution Reconstruction Method for Text Images
A technology of super-resolution reconstruction and sparse constraints, which is applied in the field of sparse-constrained adaptive NLM super-resolution reconstruction for text images, which can solve problems such as low sequence, low spatial resolution of characters in resolution images, and poor recognizability
- Summary
- Abstract
- Description
- Claims
- Application Information
AI Technical Summary
Problems solved by technology
Method used
Image
Examples
Embodiment
[0039] 1. At first introduce the theoretical method basis that the present invention relates to:
[0040]As a special image, the character image has its remarkable characteristics: First, the spatial gray density distribution of the character image has strong statistical sparsity, that is, it has obvious heavy tailing phenomenon. Secondly, the character image has strong self-similarity and structural sparsity, the same character in the image has a high probability of recurrence, and the same stroke structure between characters also has a great recurrence rate, which makes the redundant information of the character image more accurate. Many, showing a strong structural sparsity. Furthermore, the texture details of the character image are more, and it is easy to cause loss of details in the process of processing. Finally, the hierarchy of character images is highly structured, with line and character spacing largely unchanged. These characteristics of character images serve as...
PUM
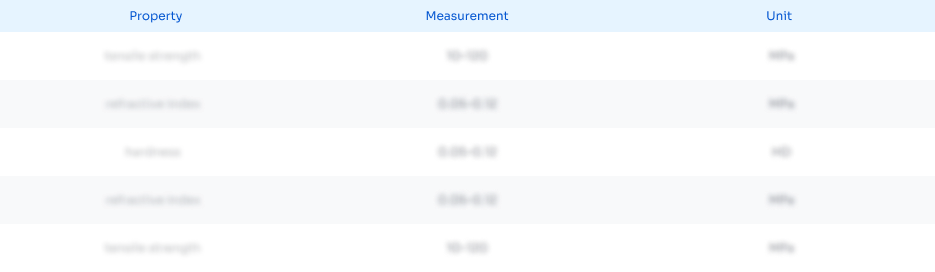
Abstract
Description
Claims
Application Information
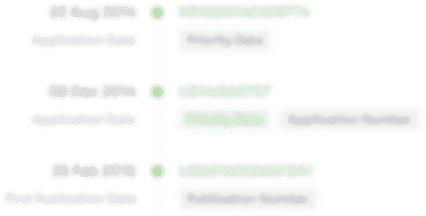
- R&D Engineer
- R&D Manager
- IP Professional
- Industry Leading Data Capabilities
- Powerful AI technology
- Patent DNA Extraction
Browse by: Latest US Patents, China's latest patents, Technical Efficacy Thesaurus, Application Domain, Technology Topic, Popular Technical Reports.
© 2024 PatSnap. All rights reserved.Legal|Privacy policy|Modern Slavery Act Transparency Statement|Sitemap|About US| Contact US: help@patsnap.com