Semi-supervised learning-based multi-gesture facial expression recognition method
A technology of facial expression recognition and semi-supervised learning, applied in the field of facial expression recognition, can solve the problems of reduced recognition rate and poor algorithm robustness
- Summary
- Abstract
- Description
- Claims
- Application Information
AI Technical Summary
Problems solved by technology
Method used
Image
Examples
Embodiment Construction
[0051] The technical scheme that the present invention takes is:
[0052] A multi-pose facial expression recognition algorithm based on semi-supervised learning. In this method, the face area of the expression image is firstly segmented by manual segmentation, and the histogram equalization method is used to compensate the illumination of the face area; then the LDA algorithm is used to extract the expression features of the image as samples. Let the training set contain samples of all frontal images and a small number of side images, and the test set contains samples of a large number of side images. Because the frontal images in the training samples are deflected relative to the test set images, the algorithm adjusts the weights to reduce the weight of misclassified samples while ensuring that the weights of correctly classified samples remain unchanged, and finally achieves The purpose of suppressing misclassified samples and improving the effect of facial expression rec...
PUM
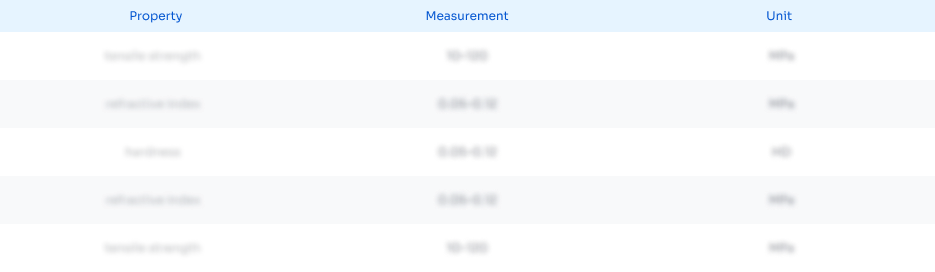
Abstract
Description
Claims
Application Information
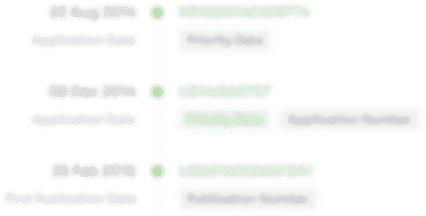
- R&D
- Intellectual Property
- Life Sciences
- Materials
- Tech Scout
- Unparalleled Data Quality
- Higher Quality Content
- 60% Fewer Hallucinations
Browse by: Latest US Patents, China's latest patents, Technical Efficacy Thesaurus, Application Domain, Technology Topic, Popular Technical Reports.
© 2025 PatSnap. All rights reserved.Legal|Privacy policy|Modern Slavery Act Transparency Statement|Sitemap|About US| Contact US: help@patsnap.com