Multi-moving-object feature expressing method suitable for different scenes
An array element and transducer technology, applied in the field of moving object recognition, can solve the problems of redundancy, low contribution, useless invariant moment value, etc.
- Summary
- Abstract
- Description
- Claims
- Application Information
AI Technical Summary
Problems solved by technology
Method used
Image
Examples
Embodiment 1
[0042] An example in the road monitoring scenario, the process is as follows figure 2 shown.
[0043] Step 1: Select {ordinary car Car, pedestrian Person, bus Bus, medium-sized bus Van, bicycle Bicycle} moving object category.
[0044] Locate the N moving objects appearing in the road surveillance video as class Class, and the class set is {CCar, CPerson, Cbus, Cvan, CBicycle}, and then extract the specific object corresponding to each class in the video, CCar class Corresponding extraction {Car1, Car2, Car3, ... Cari}, CPerson class corresponding extraction {Person1, Person2, Person3, ...Personi}, CBus class corresponding extraction {Bus1, Bus2, Bus3, ... Busi}, CVan class corresponding extraction {Van1, Van2, Van3, ...Vani}, CBicycle class corresponds to extract {Bicycle1, Bicycle2, Bicycle3, ...Bicyclei}. For each Car object, calculate various invariant moment values of its various angle forms, such as: , ,...
[0045] , other moving objects in road monitoring...
Embodiment 2
[0072] Example in the river channel monitoring scenario:
[0073] Step 1: Select {boat Boat, ordinary car Car, small crane SmallCrane, medium-sized crane Medium-sizedCrane, pedestrian Person} moving object category.
[0074]Locate the N moving objects appearing in the river channel monitoring video as class Class, and the class set is {CBoat, CCar, CSmallCrane, CMedium-sizedCrane, CPerson}, and then extract the specific objects corresponding to each class in the video, The CBoat class corresponds to extract {Boat1, Boat2, Boat3, ...Boati}, and so on, to calculate the specific objects of all categories.
[0075] For each Boat object, calculate various invariant moment values of its various angle forms, such as: , ,... Using the mean value and formula (1) to further calculate the initial input data as follows:
[0076] , , ,...
[0077] For other moving objects in river channel monitoring, refer to the calculation process of the Boat object.
[0078] The sec...
Embodiment 3
[0087] Example in Community Monitoring Scenario
[0088] Step 1: Select {Bicycle, Medium-sized Van, Ordinary Car, Pedestrian Person} sports object category.
[0089] Locate the N moving objects appearing in the community monitoring video as a class (Class), and the class set is {CBicycle, CVan, CCar, CPerson}, and then extract the corresponding specific object for each class in the video, CBicycle class Correspondingly extract {Bicycle1, Bicycle2, Bicycle3, ... Bicyclei}, and so on, to calculate the specific objects of all categories.
[0090] For each Bicycle object, calculate the various invariant moment values of its various angle forms, such as: , ,...
[0091]
[0092] Using the mean value and formula (1) to further calculate the initial input data as follows:
[0093] , , ,...
[0094] The moving objects in other cell monitoring refer to the calculation process of the Bicycle object.
[0095] The second step: use the formula (2) to calculate all th...
PUM
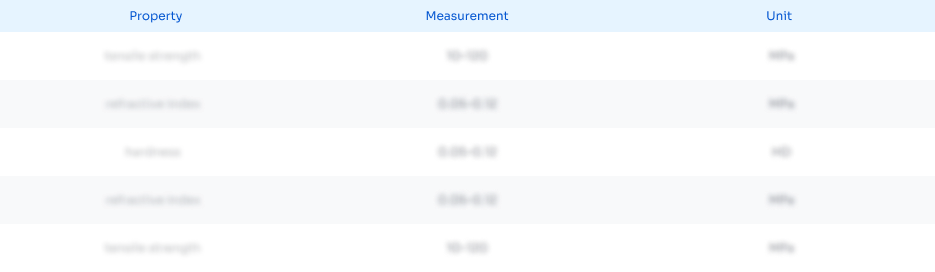
Abstract
Description
Claims
Application Information
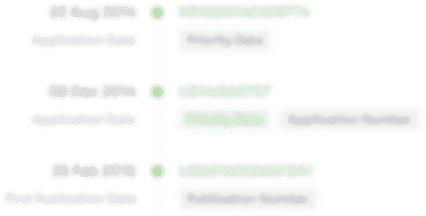
- R&D Engineer
- R&D Manager
- IP Professional
- Industry Leading Data Capabilities
- Powerful AI technology
- Patent DNA Extraction
Browse by: Latest US Patents, China's latest patents, Technical Efficacy Thesaurus, Application Domain, Technology Topic, Popular Technical Reports.
© 2024 PatSnap. All rights reserved.Legal|Privacy policy|Modern Slavery Act Transparency Statement|Sitemap|About US| Contact US: help@patsnap.com