Cluster-based collaborative filtering commodity recommendation method and system
A product recommendation and collaborative filtering technology, applied in the field of collaborative filtering product recommendation methods and systems, can solve the problems of inaccurate recommendation results and difficulty in extracting content features.
- Summary
- Abstract
- Description
- Claims
- Application Information
AI Technical Summary
Problems solved by technology
Method used
Image
Examples
Embodiment
[0063] Example: such as figure 1 As shown, we use the rating data of movielens. Movielens is a movie website. Users can rate the movies on the website after watching them. The score is 1-5. The higher the score, the more the user likes the movie. We obtained the data of 943 users in total. These users rated 100,000 times on 1,682 movie products of 18 types. In order to verify the accuracy of our recommendation results, we take out 20% of the scoring data as the test set. Our experimental result measurement method is measured by MAE mean absolute error, and the formula is as follows Here, N represents the total number of predicted scores, P i Denotes the i-th prediction score, R i Denotes the actual score of the i-th movie in the corresponding test set. The smaller the MAE, the more accurate our forecast is. In our experiments, we first investigate the effect of matrix sparsity on system recommendation performance. The sparsity is decreased by 10% and tested separately....
PUM
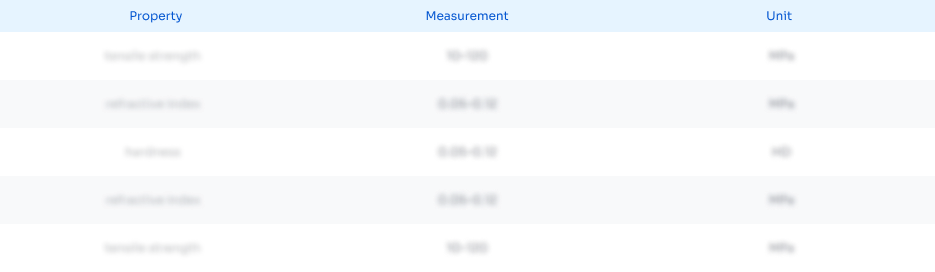
Abstract
Description
Claims
Application Information
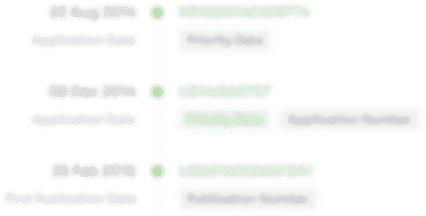
- R&D
- Intellectual Property
- Life Sciences
- Materials
- Tech Scout
- Unparalleled Data Quality
- Higher Quality Content
- 60% Fewer Hallucinations
Browse by: Latest US Patents, China's latest patents, Technical Efficacy Thesaurus, Application Domain, Technology Topic, Popular Technical Reports.
© 2025 PatSnap. All rights reserved.Legal|Privacy policy|Modern Slavery Act Transparency Statement|Sitemap|About US| Contact US: help@patsnap.com