Sentiment classification method based on multi-source field instance migration
A technology for sentiment classification and multi-source fields, applied in the field of sentiment classification based on instance migration in multi-source fields, can solve problems such as weight mismatch
- Summary
- Abstract
- Description
- Claims
- Application Information
AI Technical Summary
Problems solved by technology
Method used
Image
Examples
Embodiment Construction
[0042] combined with figure 1 It can be seen that a sentiment classification method based on multi-source domain instance migration includes the following steps:
[0043] Step 1) Introduce multi-source learning. By transferring samples from different source domains or combining the characteristics of multiple source domains, transfer learning is more stable and effective. More initial weights are assigned to target samples to alleviate weight mismatch. The problem, and resampling the data at each step to improve the phenomenon of reference imbalance;
[0044] Step 1.1), initialize the weight vector ,in for the first The weight vector of the training samples in the source domain is the weight vector of the training samples in the target domain;
[0045] Step 1.2), starting from the first iteration, calculate the total weight of training samples in the source domain, set ,in is the number of training samples for all source domains, for the first The source domain ...
PUM
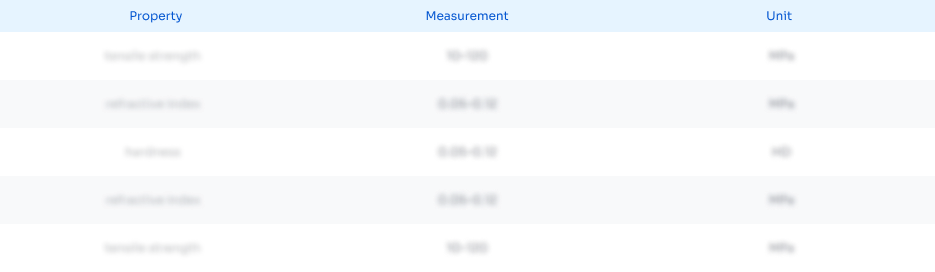
Abstract
Description
Claims
Application Information
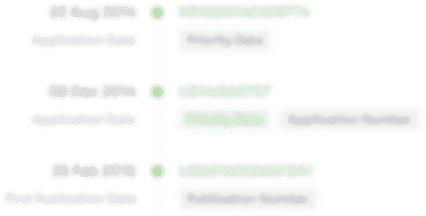
- R&D Engineer
- R&D Manager
- IP Professional
- Industry Leading Data Capabilities
- Powerful AI technology
- Patent DNA Extraction
Browse by: Latest US Patents, China's latest patents, Technical Efficacy Thesaurus, Application Domain, Technology Topic, Popular Technical Reports.
© 2024 PatSnap. All rights reserved.Legal|Privacy policy|Modern Slavery Act Transparency Statement|Sitemap|About US| Contact US: help@patsnap.com