Improved latent Dirichlet allocation-based natural image classification method
A natural image and classification method technology, applied in the field of image processing, can solve the problems of reducing classification accuracy and shortening classification time, achieve the effect of complete feature information extraction and improve average classification accuracy
- Summary
- Abstract
- Description
- Claims
- Application Information
AI Technical Summary
Problems solved by technology
Method used
Image
Examples
Embodiment Construction
[0019] Reference figure 1 The specific implementation steps of the present invention are as follows:
[0020] Step 1: Use the grid block method to perform grid dense sampling on each natural image, and obtain the corresponding grid sampling points of each natural image.
[0021] The dense grid sampling of each natural image is to divide each natural image evenly with horizontal and vertical lines to obtain each grid sampling point of each natural image.
[0022] Step 2. Use the Scale Invariant Feature Transformation (SIFT) algorithm for each grid sampling point to extract its Scale Invariant Feature Transformation (SIFT) features.
[0023] (2a) Use each grid sampling point in the natural image as a key point for generating SIFT features;
[0024] (2b) Sampling in an N×N neighborhood window centered on the key point, and using a histogram to count the magnitude of the gradient direction of the neighborhood pixels, N is an even number not less than 2;
[0025] Preferably, N=4;
[0026] (2c...
PUM
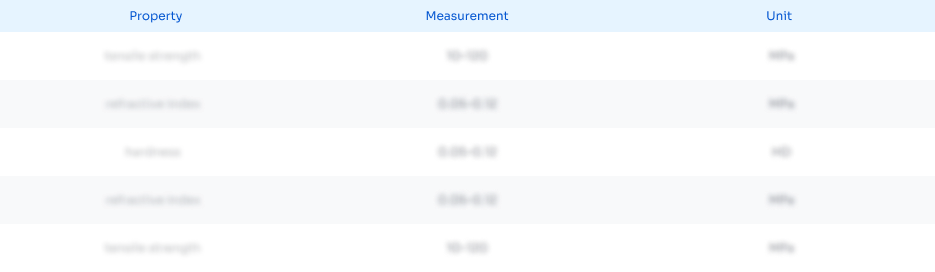
Abstract
Description
Claims
Application Information
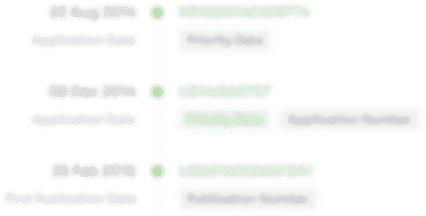
- R&D Engineer
- R&D Manager
- IP Professional
- Industry Leading Data Capabilities
- Powerful AI technology
- Patent DNA Extraction
Browse by: Latest US Patents, China's latest patents, Technical Efficacy Thesaurus, Application Domain, Technology Topic, Popular Technical Reports.
© 2024 PatSnap. All rights reserved.Legal|Privacy policy|Modern Slavery Act Transparency Statement|Sitemap|About US| Contact US: help@patsnap.com