Automatic grouping multi-scale lightweight deep convolutional neural network optimization method
A convolutional neural network and neural network technology, applied in the field of automatic grouping multi-scale lightweight deep convolutional neural network optimization, can solve the problems of high difficulty in crossover process design and insufficient diversity of offspring, etc. Effects of portability and scalability, performance and efficiency improvements, network performance advantages
- Summary
- Abstract
- Description
- Claims
- Application Information
AI Technical Summary
Problems solved by technology
Method used
Image
Examples
Embodiment Construction
[0048] The method of the present invention will be specifically described below in conjunction with the accompanying drawings.
[0049] Such as image 3 As shown, an automatic grouping multi-scale lightweight deep convolutional neural network optimization method, the specific steps include:
[0050] Step 1, use the symmetric sine-cosine algorithm to perform evolutionary search to obtain the population to be evaluated;
[0051] The specific method of step 1 is:
[0052] Step 1.1: Population initialization. Aiming at the grouping ratio problem in the multi-scale lightweight convolution module to be determined, encode the individual of the population in the evolutionary algorithm, and set the initial fitness value of the individual to 0, and randomly initialize the most good individual
[0053] Step 1.1.1: Input the population size N, the individual dimension D, here set the value of N to 20, the value of D to 8, set the value of the initial individual counter to 0, and use th...
PUM
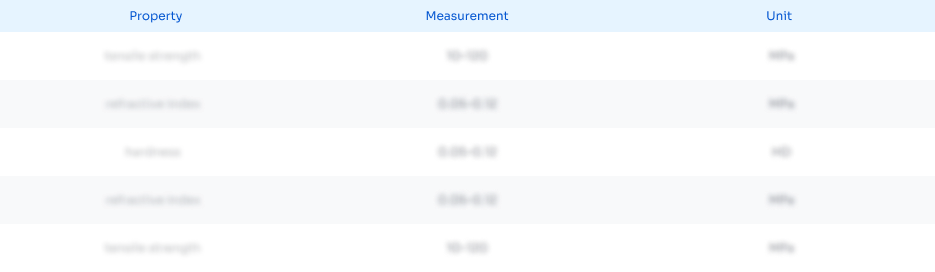
Abstract
Description
Claims
Application Information
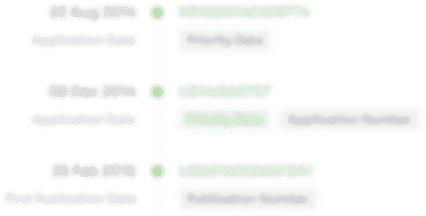
- R&D Engineer
- R&D Manager
- IP Professional
- Industry Leading Data Capabilities
- Powerful AI technology
- Patent DNA Extraction
Browse by: Latest US Patents, China's latest patents, Technical Efficacy Thesaurus, Application Domain, Technology Topic, Popular Technical Reports.
© 2024 PatSnap. All rights reserved.Legal|Privacy policy|Modern Slavery Act Transparency Statement|Sitemap|About US| Contact US: help@patsnap.com