Polarimetric SAR image classification method based on semi-supervised SVM and mean shift
A classification method and semi-supervised technology, applied in the field of image processing, to solve the difficulty of marking and improve the effect of poor adaptability
- Summary
- Abstract
- Description
- Claims
- Application Information
AI Technical Summary
Problems solved by technology
Method used
Image
Examples
Embodiment 1
[0048] Embodiment 1, in conjunction with attached figure 1 describe.
[0049] The implementation method step of the present invention is as follows: a kind of polarization SAR image classification method based on semi-supervised SVM and MeanShift comprises the steps:
[0050] (1) Establish polarimetric SAR image classification training set Tr and image classification test set Ts respectively;
[0051] (1a) Randomly select n samples from the low entropy H 0.8 of the polarimetric SAR image to form the polarimetric SAR image classification training set Tr, and the remaining samples form the polarimetric SAR image classification training set Tr. SAR image classification test set Ts, the total number of samples is A, if the amount of data is large, the image classification test set Ts can be divided into 80×80 small blocks and processed sequentially, where the value range of n is any integer between 50-200 ;
[0052] (2) Input the samples of the polarization SAR image classific...
Embodiment 2
[0062] Embodiment 2, in conjunction with attached Figure 1-5 describe.
[0063] On the basis of embodiment 1, the steps described in step (1a) in the above image classification step are randomly selected from the low entropy H0.8 of the polarimetric SAR image respectively n samples constitute the polarization SAR image classification training set Tr, and the remaining samples constitute the polarization SAR image classification test set Ts, the total number of samples is A, if the amount of data is large, the image classification test set Ts can be divided into 80×80 small The blocks are processed sequentially, where the value range of n is any integer between 50-200, and the entropy of each sample is calculated according to the following formula,
[0064] H = - Σ i = 1 3 p i lo g ...
PUM
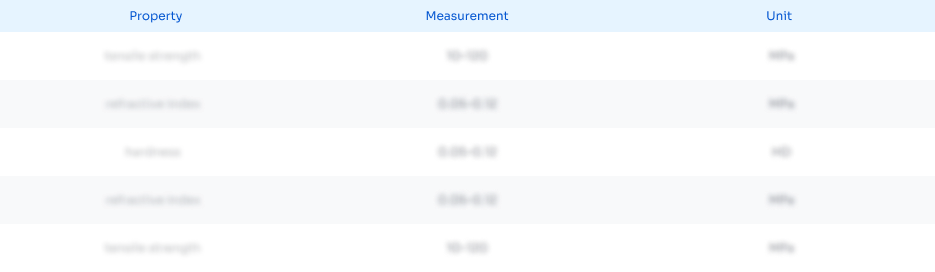
Abstract
Description
Claims
Application Information
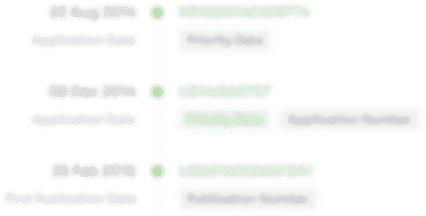
- R&D Engineer
- R&D Manager
- IP Professional
- Industry Leading Data Capabilities
- Powerful AI technology
- Patent DNA Extraction
Browse by: Latest US Patents, China's latest patents, Technical Efficacy Thesaurus, Application Domain, Technology Topic, Popular Technical Reports.
© 2024 PatSnap. All rights reserved.Legal|Privacy policy|Modern Slavery Act Transparency Statement|Sitemap|About US| Contact US: help@patsnap.com